Welcome to our weekly news post, a combination of thematic insights from the founders at ExoBrain, and a broader news roundup from our AI platform Exo…
Themes this week
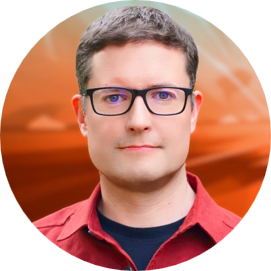
JOEL
This week we look at:
- OpenAI’s latest agent that can synthesise complex research in minutes.
- The big tech companies committing a combined $320 billion to AI infrastructure in 2025.
- Stanford researchers who demonstrate how to train a reasoning model for less than $50.
Deep Research shows the way for agents
On a low-budget live stream from the OpenAI offices in Japan on Sunday night, OpenAI unveiled Deep Research, its second ‘agent’ offering of 2025. Despite the modest event, CEO Sam Altman stated that this could potentially do “a single-digit percentage of all economically valuable tasks in the world”. Deep Research is a ChatGPT-based AI tool designed to autonomously conduct complex web-based research from an initial prompt and then some clarifying questions. OpenAI claims it “accomplishes in tens of minutes what would take a human many hours” but will only initially be available for the $200/month pro users.
The technical backbone of Deep Research is OpenAI’s as yet unreleased o3 reasoning model, one of a new class of AI models (which also includes DeepSeek’s R1 and Google’s Gemini 2.0 Flash Thinking) designed to think more deeply and methodically. According to OpenAI, this model was trained via ‘reinforcement learning’ on hard web browsing and data analysis tasks. It plans multi-step research, adapts as it uncovers information, and even backtracks when it hits dead ends. This combination of reasoning and orchestration of tasks like browsing, extracting content, and chaining together analysis steps demonstrates the future of agentic AI (and perhaps knowledge work and economic activity in general).
ExoBrain went to work testing it extensively, and it’s safe to say that this is the most powerful AI agent the world has seen, at least as of this week.
Key strengths:
- Making sense of multiple sources, reducing the need for lengthy human reasoning and cross-referencing and hundreds of open browser tabs!
- Generating almost complete, well referenced reports on a topic that through careful prompting can include all the desired sections, tables and conclusions in a single step.
- The ability to continue to interrogate the output with the model you started the chat with. For example, if you invoke the Deep Research feature from within an o1 chat, you can continue to use that powerful reasoning model and question and challenge the research.
- Superior to Google’s Deep Research tool in that it provides much greater levels of insight and synthesis rather than being simply an information gathering tool.
Key weaknesses:
- Although citations are provided, it can still misinterpret or misrepresent data, meaning critical outputs require careful human verification.
- Sometimes the sources used feel limited, and this research will only be as good as the available web information. In general, the system sometimes struggles to distinguish between authoritative sources and less reliable or biased information. A means to grade or classify sources would help greatly.
- No access to paywalled content and non-public information, and it will be a major step forward users can enter subscription details or run over their corporate knowledge bases.
- Varied quality, likely driven by the availability of information and in some cases the quality of the prompt. Not every output feels expert quality.
- A limit of 100 searches a month reflects the amount of compute that’s needed, but we envisage this will drop, especially as smaller models will ultimately be improved to pick up where the expensive state-of-the-art models are currently needed.
- Ultimately the output is a giant chunk of text that while valuable still needs further processing. The next stage will be to work out what you do with 45 pages of information on a topic… what is valuable, what can be archived, and currently the Deep Research process is not as configurable it will need to be to deliver truly actionable end-product.
We found in general that Deep Research struggles with time based factual correctness (think latest pricing, product feature sets, or the current Manchester United squad where a set of web pages would provide conflicting information from different points in time. Whereas the agent performs best with complex case studies and analysis that require weighing multiple strategies. For example, when examining startup valuation approaches, it can explore various methodologies, consider market conditions, and assess different expert opinions. Here, absolute factual correctness matters less than building a comprehensive view of different evolving paths based on solid insights form information on the web.
Here are our top tips for getting the most from Deep Research:
- Much like with a Google search, use clear keywords and well-known technical terms. Deep Research picks these up to guide its web searches, so using precise terminology (like specific product names or technical concepts) helps it find relevant information faster.
- Upload context files and text before you invoke the research. If you’re researching a complex topic, providing info upfront helps guide the agent and fills knowledge gaps it might encounter, or support decisions based on the actual context.
- Specify your output format. Tell Deep Research exactly how you want the information presented, the sections, structure, narrative, language, formatting etc. This saves time on reformatting 45 pages later!
- Respond to clarifying questions in detail. When Deep Research asks for clarification, providing clear, specific answers helps it stay on track and avoid wasted compute time.
- Break complex queries into steps. Instead of asking for everything at once, consider splitting your research into logical phases. As with any AI tool, starting too broad leads to generic outputs, and too narrow can mean the output is too specific and is not leveraging the strengths of the system.
- Use a model like o1 or Claude to ‘craft’ your research prompt in advance. Explain to that model the intention, maybe even share these top tips, and you can make the most of the limited Research runs.
- Try to trigger the agent’s ability to do deeper analysis across multiple dimensions. The key is moving from a “what is happening” prompt to “what does it mean and what are the different ways forward” structure. This plays to Deep Research’s strengths in processing multiple sources and drawing connections, rather than just fact-finding. (For basic fact finding a combination of Grok 2 on X, and now DeepSeek R1 or o3 on Perplexity AI are much faster and probably more reliable. You could for example include outputs from those tools in the chat before you invoke Deep Research to augment its thinking.
The launch of Deep Research appears to be just the beginning of OpenAI’s expansion into purpose-built AI agents. Recent announcements and leaks point to a range of specialised tools in development. A new B2B Sales Agent aims to streamline the sales process by enriching lead data, checking calendars and drafting meeting requests. Meanwhile, a Software Engineering Agent (SWE), is being rumoured, that will handle tasks typically managed by mid-level developers – from coding and debugging to project planning. These join existing projects like Operator, which handles general tasks such as appointment booking or app configuration and testing. Its not hard to see the spine of a business capability that start from research, to planning, to sales, to software product building to deployment and customer service. OpenAI is looking to precipitate future reasoning models combined with specialised agent-based orchestrations to drive the use of its ecosystem.
To give a sense of speed of progress, 2 weeks ago a benchmark entitled “Humanity’s Last Exam” was released to put AI tools to the test with 3,000 hard questions. Last week the highest scoring was o3-mini with 13.0%. This was doubled by Deep Research in short order, with 26.6%.
Takeaways: Expect gradual expansion of Deep Research to other subscriptions tiers and broader geographical availability, alongside efforts to reduce its compute footprint. At the same time, we will also see many variants of this using other reasoning models such as from Google or DeepSeek. Deep Research is most valuable for tasks requiring synthesis of multiple viewpoints rather than simple fact-finding. Users should turn to it for complex analysis and literature reviews, while keeping simpler tools for basic factual queries. The technology is still new and imperfect, but its arrival in early 2025 will be remembered as a milestone. It’s a preview of a future where for better or worse, much of the heavy lifting in intellectual labour will be offloaded to AI agents. And as OpenAI and others race forward, the challenge will be ensuring these agents are reliable, transparent, and used in ways that truly empower humankind.
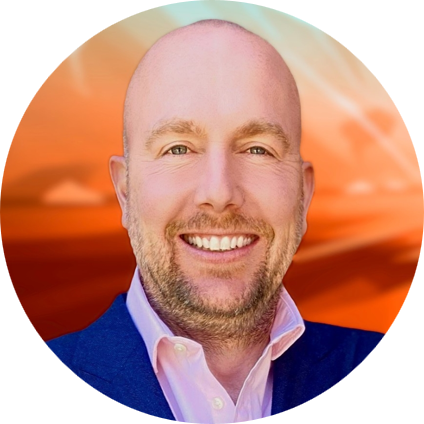
JOOST
Big tech spending hits new heights
ExoBrain updated our market view slide deck this week, increasing the eye-watering numbers summarising the billions to be deployed in AI data centre capital expenditure in 2025. The commitments have reached new heights, with Amazon, Microsoft, Google, and Meta forecasting a combined $320 billion spend – up 30% from 2024’s record $246 billion.
But is this bold spending justified? The case for investment is compelling. By building out massive AI infrastructure now, these companies aim to cement their leadership in cloud computing and AI services. Historical precedent suggests such ambitious tech investments often yield substantial long-term returns.
Meta’s success offers a blueprint. While its heavy AI spending raised eyebrows initially, improved ad targeting has already shown clear returns. Meanwhile, Amazon leads the new wave with a $100 billion commitment, while Microsoft and Google each plan around $75-80 billion.
Yet risks loom. Microsoft and Alphabet each lost $200 billion in market value after announcing their plans. Every dollar spent on data centres is one not returned to shareholders or invested in other innovations.
Takeaways: While the scale of investment might seem excessive, the alternative – getting left behind in the AI race – likely poses an even greater risk. As we set out in other articles this week, not having the capacity to serve the agentic AI workloads that will design and build new products and drive business growth and productivity for the world’s businesses is inconceivable for the mag 7. The next few quarters will be crucial in validating these bold bets.
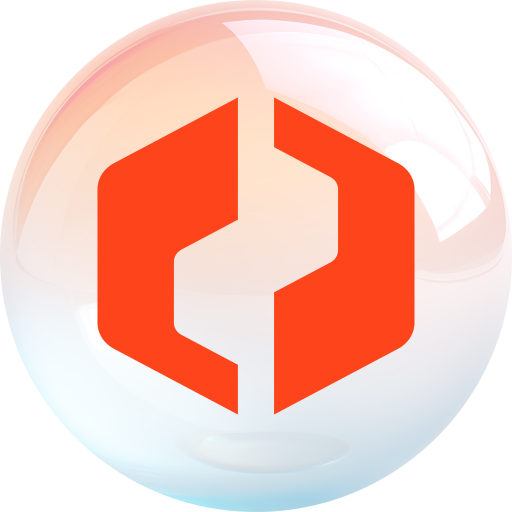
EXO
How to create a reasoning model for $50
Over the last few months, we’ve covered o1, o3, DeepSeek R1-lite and R1, and Gemini 2.0 Flash Thinking as models which corroborate the central trend in recent AI development. Specifically, they show that if you train a model on examples of long-form reasoning (think topics such as maths and coding) and then give it more time to think at point of use, it can get way smarter.
This week a team from Stanford released a paper that demonstrated this in a pure form. They curated a set of just 1,000 reasoning examples and for less than $50 extracted from Google Gemini 2.0 Flash Thinking and used them to train a base model (Alibaba’s Qwen 2.5), and then implemented a neat trick to make the model think for longer. They appended a “wait” command at the end of each response to make it keep going. To keep it thinking longer, they added this several times. This combination of techniques increased the models benchmark scores dramatically.
You can see on the right chart here, along the x-axis more wait and think loops increased its ability to answer university level questions. The research shows you don’t need massive datasets or complex training methods to achieve this ‘test-time scaling’ effect – you just need high-quality examples and extended reasoning time. With OpenAI hiring PhDs and paying then $100 an hour to write out answers to complex problems, it’s not surprising Sam Altman stated at an event in Germany today that he sees no limit to where these models can go.
Weekly news roundup
AI business news
- Exclusive: OpenAI co-founder Sutskever’s SSI in talks to be valued at $20 billion, sources say (Indicates the massive valuations and investor interest in AI safety initiatives)
- Microsoft is forming a new unit to study AI’s impacts (Shows big tech taking proactive steps to understand AI’s societal effects)
- OpenEuroLLM: Europe’s new initiative for open-source AI development (Represents Europe’s push for AI sovereignty and open innovation)
- AVAXAI brings DeepSeek to Web3 with decentralised AI agents (Shows convergence of AI with blockchain technology)
- ‘Seismic impact’ of AI revolution could see 70% computer-based jobs replaced (Critical insight into AI’s potential impact on knowledge work)
AI governance news
- UK makes use of AI tools to create child abuse material a crime (Shows governments addressing AI misuse with specific legislation)
- Elon Musk’s DOGE is working on a custom chatbot called GSAi (Demonstrates growing intersection of crypto and AI development)
- Exclusive: Brits want to ban ‘smarter than human’ AI (Reveals public sentiment towards AI regulation)
- Google is adding AI watermarks to photos manipulated by Magic Editor (Shows progress in AI content authentication)
- OpenAI launches data residency in Europe (Important for European businesses requiring local data processing)
AI research news
- SmolLM2: When Smol goes big — data-centric training of a small language model (Advances in efficient AI model training)
- Boosting multimodal reasoning with MCTS-automated structured thinking (Important progress in AI reasoning capabilities)
- VideoJAM: Joint appearance-motion representations for enhanced motion generation in video models (Breakthrough in AI video generation)
- OmniHuman-1: Rethinking the scaling-up of one-stage conditioned human animation models (Advances in human animation AI)
- Thoughts are all over the place: On the underthinking of o1-like LLMs (Critical analysis of current LLM limitations)
AI hardware news
- UAE to invest billions building new AI data centre in France (Shows global investment in European AI infrastructure)
- Amazon expects to spend $100 billion on capital expenditures in 2025 (Indicates massive investment in AI infrastructure)
- AI chip firm Cerebras partners with France’s Mistral, claims speed record (Shows progress in European AI computing capabilities)
- Trump committed to chip tariffs despite Nvidia CEO meeting (Political implications for AI hardware supply chains)
- Top Taiwan chip designer MediaTek running simulations for possible US tariffs (Impact of trade policies on AI chip industry)