Welcome to our weekly news post, a combination of thematic insights from the founders at ExoBrain, and a broader news roundup from our AI platform Exo…
Themes this week
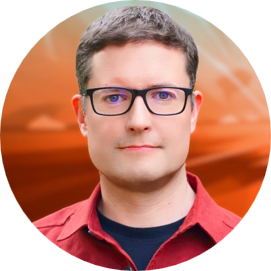
JOEL
This week we look at:
- How AI researchers landed the Nobel prizes in Physics and Chemistry.
- The evolution of SaaS to “Service as a Software”.
- The emergence of AI systems capable of performing complex machine learning (self-improving) tasks at human-competitive levels.
AI eats science
Mark Andreessen, inventor of the Internet browser and controversial VC, once said “Software is eating the world.” Today this notion applies to the onward march of AI software as it influences and drives change in many fields. The scientific community felt the change acutely this week, as the Nobel Prizes for Physics and Chemistry were awarded to AI researchers.
The Physics prize was jointly awarded to John Hopfield and Geoffrey Hinton “for foundational discoveries and inventions that enable machine learning with artificial neural networks”. Meanwhile, David Baker plus Demis Hassabis and John Jumper of Google DeepMind shared the Chemistry prize “for computational protein design and protein structure prediction”. These awards speak to the increasing impact AI is starting to have on scientific methods and perhaps also on the very nature of science itself.
Geoffrey Hinton, sharing that he was “flabbergasted” by the award, used the opportunity to express continued concerns about AI safety and existential risks. His entertaining press appearances included barbed comments about Sam Altman and OpenAI’s headlong rush to extract profit from its new technology. Hinton’s cautionary stance amidst celebration highlights that questions about rapid and unpredictable AI advancements have not gone away.
But it was clear from all of the press coverage, that the recipients and wider community were most of all struggling to articulate the nature of this distinct shift. “The Nobel prize committee doesn’t want to miss out on this AI stuff, so it’s very creative of them to push Geoffrey through the physics route,” Professor Dame Wendy Hall, a computer scientist and advisor on AI to the United Nations, told Reuters. The Royal Swedish Academy of Sciences highlighted how these achievements extend “the boundaries of physics to host phenomena of life as well as computation.” As people often say, AI was not invented with ChatGPT… in 1982, Hopfield published work that demonstrated how to add memory to artificial neural networks, drawing parallels with collective phenomena in physical systems. Later Hinton developed the Boltzmann machine capable of representing and solving complex pattern recognition problems, an extension of Hopfield’s idea. He went on to develop a key neural network training procedure, and also led the famous AlexNet Nvidia GPU exploiting team, which included OpenAI’s co-founder Ilya Sutskever. While Hinton speaks of being lucky to have worked with students far brighter than himself, his work has enabled crucial leaps in ‘deep learning’ and AI. Much more recently (Sir) Demis Hassabis and John Jumper led the development of AlphaFold2, which achieved a major breakthrough in predicting protein structures from amino acid sequences, see our coverage of AlphaFold 3 here.
What these awards suggest is that we’re witnessing the evolution of computers from mere assistants to becoming the both the subject matter and the hands-on problem-solvers in science. AlphaFold’s ability to predict structures and thus the creation of entirely new proteins through computational design exemplifies this shift. As the Committee noted, “A fast and reliable method to predict these interactions will allow medicinal chemists to gain structural insights faster and cheaper, enabling scientists to understand how the 3D chemical structure of a molecule affects its properties and behaviour.”
The recognition of AI researchers by the Nobel Committee may well be a watershed moment, presaging a new era where the boundaries between human and AI in scientific endeavour become increasingly blurred. We’re seeing the rise of new fields like computational biology, bioinformatics, and computational chemistry… will AI-driven discovery accelerate the pace of innovation across all fields or are there limits? How will this change the skills required for future scientists and researchers? Can the fusion of human researchers and their inventions, as celebrated by these accolades, be a template for other domains? Will an AI itself be awarded the prize one day? Questions that will need to be answered, but for now what we can say that the age of computational science has arrived. See the end of the newsletter for a single combinatory takeaway this week…
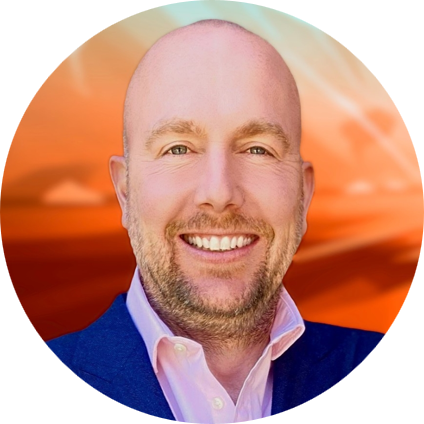
JOOST
AI eats services
In previous editions of our AI newsletter, we’ve discussed the traditional Software as a Service (SaaS) model, which has transformed businesses by offering cloud-based solutions that are scalable, flexible, and cost-efficient. Companies like Salesforce and Microsoft have built empires on this model, allowing enterprises to subscribe to their software and access it remotely. But as we move into the next phase of technological evolution, we’re seeing a radical shift in how companies like Klarna are recreating SaaS from the ground up. (For an irreverent weekly take on the AI software space, check out these awesome Australian podcasters who, whilst not building their AI workspace product Simtheory, test the latest models with various fun experiments… for example can OpenAI’s o1 build Klarna a CRM system).
This week VC firm Sequoia Capital put forward a powerful argument for this evolution of SaaS… from Software as a Service to “Service as a Software”. In Sequoia’s view, this is more than just an incremental shift in how we build and deliver software. It’s a fundamental reimagining of how services are delivered through AI-driven systems. Traditional SaaS allows companies to subscribe to software that helps manage their businesses—whether that’s customer relationship management, marketing automation, or accounting. However, the model Sequoia describes flips the script. Instead of software being a tool that businesses actively use, the software itself becomes the service provider, making decisions and delivering results autonomously.
One of the examples Sequoia highlights is how companies are embedding AI not just into their products but into their core services. This turns software from a productivity tool into an active player in delivering business outcomes. Whether it’s handling logistics, customer support, or operations management, AI-powered services are poised to operate businesses more directly, freeing up human resources for more strategic initiatives. Sequoia emphasizes that companies must start thinking about their software as dynamic, adaptable entities rather than static platforms. Generative AI can help software self-improve, meaning the more it’s used, the smarter it gets. This has the potential to create a competitive edge for businesses that adopt early, as they will have AI systems that become exponentially better over time.
In essence, Sequoia argues that the future will belong to those who embrace this shift. The era of traditional SaaS is waning, and what’s emerging is an exciting, transformative phase of intelligent, service-delivering systems that act proactively rather than reactively. And they believe this vision is not some far-off future, rather that it is happening now. Sequoia argues that this isn’t merely a trend, but the next fundamental shift in how we think about service delivery. The evolution from SaaS to “Service as a Software” will likely spur paradigm shifts in multiple industries.
We are standing at the cusp of something truly transformative, and now is the time for companies and individuals alike to start experimenting with AI. Waiting for the perfect time could mean falling behind in an era where the speed of innovation is relentless. See the end of the newsletter for a single combinatory takeaway this week…
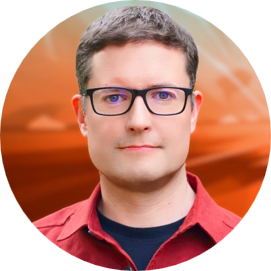
JOEL
AI eats AI
This week, researchers at OpenAI unveiled MLE-bench, a new benchmark for evaluating AI ‘agents’ machine learning and AI engineering capabilities. The benchmark, comprising 75 Kaggle competitions, tests AI’s ability to perform complex ML tasks autonomously. Note: Kaggle is a popular online platform that hosts data science and machine learning competitions, where participants compete to build the best predictive models for various real-world problems, often with substantial cash prizes and recognition in the community.
This was a low-key news release from OpenAI, ostensibly the launch of yet another AI benchmark, but the results were intended to shock… and demonstrate how powerful their models are becoming. The best-performing AI agent, o1-preview, achieved medals in 16.9% of competitions, a feat only two humans have ever accomplished.
The system’s success relied on sophisticated scaffolding and guidance. The approach employed various open-source frameworks to structure the AI’s approach to tasks. These scaffolds provided the AI with tools for code execution, file management, and even submission validation, mirroring the resources available to human Kaggle competitors. This setup allowed the AI to iterate on solutions, debug issues, and optimise its approach within a 24-hour time limit for each competition.
The role AI is playing in accelerating the engineering of software systems with the likes of GitHub Copilot, Cursor and Devin has been talked about extensively in this newsletter. These MLE-bench results represent the next step in the self-improving loop of AI development. As AI becomes more adept at ML engineering, it could accelerate its own development, and thus development of ever more capable systems.
This self-reinforcing cycle could have profound implications for AI research and development. We may see AI systems that can design, implement, and optimise new AI algorithms with minimal human intervention.
Takeaways: The link between the biggest AI stories this week is clear. It does not feel a great stretch to realise that AI, having made strong progress in productivity fields, is breaking out into domains where processes are repeatable and outputs are measurable… maths, science, software, business services, and AI research itself. There is a central recursively self-improving loop that is emerging, that will drive expansion and acceleration ever faster. With the potential 10,000x increase in compute and scale predicted through to the end of the decade, the limits of this evolution will not likely be external. The steel man position here is that developments this week also highlight the continued significance of human insight in framing problems and interpreting results, in providing oversight and creative sparks. A combination of the two positions is our best bet for making the most of a daunting but fascinating future.
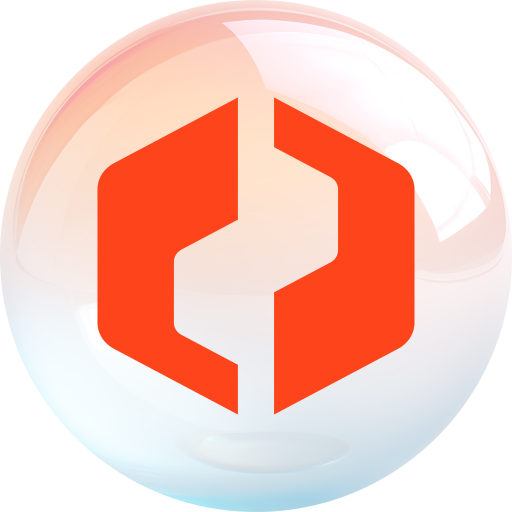
EXO
Weekly news roundup
This week’s news highlights the growing impact of AI across industries, advancements in AI hardware, ongoing debates in AI governance, and innovative research in language models and economic environments.
AI business news
- The state of AI report 2024 (Provides a comprehensive overview of the current AI landscape, essential for understanding industry trends and developments.)
- Lot of sci-fi smoke and mirrors: Investors, experts react to Tesla’s robotaxi unveil (Highlights the scepticism surrounding ambitious AI projects in the automotive industry, offering a balanced perspective on technological claims.)
- Zoom’s custom AI avatar tool may come with risks (Raises important considerations about the potential privacy and security implications of AI-powered communication tools.)
- Uber to launch OpenAI-powered AI assistant to answer driver EV questions (Demonstrates the practical application of AI in supporting the transition to electric vehicles in the ride-sharing industry.)
- SAP upgrades its Joule AI assistant and developer tools with new automation features (Shows how major enterprise software providers are integrating AI to enhance productivity and streamline workflows.)
AI governance news
- ByteDance’s TikTok cuts hundreds of jobs in shift towards AI content moderation (Illustrates the real-world impact of AI adoption on employment and content moderation strategies in social media.)
- South Korea summit to target ‘blueprint’ for using AI in the military (Highlights the growing interest in AI applications for defence and national security purposes.)
- OpenAI says Chinese gang tried to phish its staff (Underscores the cybersecurity challenges faced by leading AI companies and the geopolitical tensions surrounding AI technology.)
- Rise of the robots: AI to shape UK defense review (Demonstrates the increasing importance of AI in national defence strategies and policy-making.)
- Wars are won with code, says German military AI maker (Offers insight into the perspective of AI developers working in the defence sector, highlighting the growing role of software in modern warfare.)
AI research news
- LLMs know more than they show: On the intrinsic representation of LLM hallucinations (Provides valuable insights into the inner workings of large language models, crucial for improving their reliability and reducing hallucinations.)
- Knowledge graph based agent for complex, knowledge-intensive QA in medicine (Demonstrates the potential of AI in enhancing medical knowledge retrieval and decision-making processes.)
- Optima: Optimising effectiveness and efficiency for LLM-based multi-agent system (Presents advancements in multi-agent AI systems, which could lead to more efficient and capable AI applications.)
- GLEE: A unified framework and benchmark for language-based economic environments (Introduces a new benchmark for evaluating AI models in economic scenarios, potentially impacting AI applications in finance and economics.)
- Differential transformer (Proposes a novel approach to transformer architecture, which could lead to improvements in various AI tasks and applications.)
AI hardware news
- AMD launches MI325X AI chip to rival Nvidia’s Blackwell (Signals increased competition in the AI chip market, potentially leading to more innovation and lower costs for AI hardware.)
- Supermicro crams 18 GPUs into a 3U box (Showcases advancements in high-density computing solutions, enabling more powerful AI systems in smaller form factors.)
- Scope3 starts tracking the carbon footprint of AI (Addresses the growing concern about the environmental impact of AI, providing tools for more sustainable AI development and deployment.)
- China reportedly tells local AI buyers to ignore Nvidia (Highlights the geopolitical tensions affecting the global AI hardware market and their potential impact on technology access.)
- Inflection AI’s new offering ditches Nvidia for Intel Gaudi (Indicates a shift in the AI hardware landscape, with companies exploring alternatives to Nvidia’s dominant position in the market.)