Welcome to our weekly news post, a combination of thematic insights from the founders at ExoBrain, and a broader news roundup from our AI platform Exo…
Themes this week
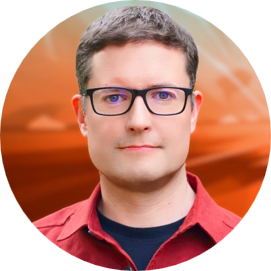
JOEL
Themes this week:
- New research from Epoch on compute limits between now and 2030.
- Google DeepMind employees and their concerns highlighting the use of AI in warfare.
- The future of productivity.
Super-size my training run
This week, research outfit Epoch published an analysis exploring AI compute growth towards 2030. Their findings suggest many thousand-fold increases are possible, but not without constraints.
At ExoBrain, we focus on three key drivers of AI progress: compute, data, and algorithms. Compute powers the training of ever-larger and more numerous models. Data fuels these models with knowledge. Algorithms determine how effectively models learn and operate. Epoch’s analysis aligns with our view, deep diving into the compute aspect and its potential constraints.
Epoch outlines several trajectories for AI scaling. The most constrained scenario, hampered mainly by energy supply limitations, suggests 5,000 to 150,000-fold increases (from GPT-4 scale AI levels) by 2030. Their upper bound, limited mostly by the fundamentals of data transfer and latency, envisions a 10-million-fold increase. The Epoch team’s central conclusion is striking “…by 2030 it will be very likely possible to train models that exceed GPT-4 in scale to the same degree that GPT-4 exceeds GPT-2 in scale” (a 10,000-fold increase).
But power constraints loom large. Bigger systems will require a leap from current 1-5 GW single location facilities to 40+ GW through distributed systems, potentially achievable by Google who have a network of datacentres across the US. Chip manufacturing is another near-term constraint. TSMC and others could theoretically ramp up to produce hundreds of millions of H100-equivalent GPUs, but this will require continued expansion of semiconductor fabrication capacity. Data availability presents another hurdle, with 2030 models potentially requiring up to 20 quadrillion effective tokens for training, more than most can envisage securing in the near-term.
Epoch also describes a “latency wall” concept, the ultimate constraint. As models grow, the minimum time to process a single datapoint increases. They estimate this would kick in at the largest scales of AI systems, highlighting the need for innovative solutions in model design and hardware architecture. This latency accumulates across the model’s layers and training iterations, potentially setting an upper bound on model size and training data for a given timeframe.
Individual training runs are also going to hit some limits in terms of hard cash. Recent run investments illustrate the trajectory, and also the financial headwinds for individual companies no matter how big. GPT-4, trained in 2022 by OpenAI on Microsoft’s kit, cost an estimated $100 million and used 2e25 FLOP (floating point operations; the measure for the calculations utilised). Two years later, Llama 3.1 required $600 million and 3.8e25 FLOP. Extrapolating this trend paints a startling picture for future costs at the frontier. By 2025, we might see GPT-5+ models with $2 billion price tags. Come 2028, a frontier model like GPT-6+ could cost $20 billion, a notable step up from the OpenAI training budget for 2024 of some $3 billion. Epoch’s 2030 projection suggests a staggering $125-250 billion for a technically feasible 2e29 FLOP training run. Such a run would be approximately 5 times Meta’s entire annual R&D budget
Takeaways: This exponential growth aligns with our ExoBrain view that AI capabilities will continue to advance rapidly, driven by massive compute increases. But this surge clearly won’t all be consumed by a few labs or big tech firms alone. What makes this journey exciting is the uses and applications that will be unlocked for the many and that we have yet to imagine. This new research is important reading for anyone wanting to understand the underlying maths, when pondering the many arguments about future AI trajectories. When the broader picture on compute is examined, limits certainly exist but there appears to be plenty of headroom for the next few years at least.
DeepMind’s military dilemma
The rapid advancement of AI in military applications was in the news this week. As conflicts in Ukraine and the Middle East accelerate the adoption of AI-powered systems, the world is facing the reality of a dual-use technologies and their potential impact on global security. Advancements in autonomous systems, learning, and vision open doors for ground breaking commercial applications, but also promise profitable opportunities in defence contracts, which is where the ethical questions kick-in.
This week it emerged that around 200 Google DeepMind employees, representing about 5% of the division have signed a letter that called for an end to the company’s contracts with military organisations, particularly citing Project Nimbus, a cloud computing contract with the Israeli military. The employees expressed worry that Google’s AI technology could be used for warfare, highlighting reports that the Israeli military uses AI for mass surveillance and targeting in Gaza. This internal dissent at one of the world’s leading AI research companies underscores a growing tension between technological advancement and geo-politics.
The US Department of Defence’s Replicator program, announced earlier this year, aims to field autonomous systems at scale within 18-24 months, underscoring the urgency of major powers pursuing military AI capabilities. This push comes as China maintains its dominance in the global drone market, with DJI dominating. A future shaped by drone warfare appears to be increasingly likely as both China and the United States build up significant armies of these unmanned aerial vehicles.
The boundaries between military and commercial AI applications are blurring. Eric Schmidt, former Google CEO, has launched White Stork, a startup developing AI-powered attack drones for Ukraine. Ukraine has become the premier testing ground for the west’s techno-military industrial complex. AI is being used for clearing minefields to enhancing drones for reconnaissance and targeted strikes. However, the conflict has also highlighted the downsides of digital weapons, including the vulnerability of AI systems to electronic warfare (EW). Recent reports suggest that China has developed new AI-powered EW chips that enable faster data analysis and improved perception capabilities at lower costs. These advancements allow Chinese military systems to detect, lock on, decode, and suppress enemy signals quickly and effectively, while maintaining smooth communication for their own forces. As concerns grow over conflict in the South China Sea, some suggest that AI’s primary would be in quickly finding targets within a hugely cluttered background, while simultaneously attempting to fool enemy AI systems.
The Ukrainian GIS Arta system exemplifies an augmented approach to battlefield decision making, offering methods for target selection described as the “Uber for artillery”. This system has notably improved Ukraine’s ability to respond across a huge geographical area. But AI decision-support also introduces risks. “We’ve learned the hard way that there is inherent human bias built into the AI system… leading to maybe misinformation being provided to the decision-maker.” – says Mallory Stewart, Assistant Secretary of State for the Bureau of Arms Control, Deterrence and Stability.
Takeaways: The integration of AI into military operations is now an undeniable reality. This brings with it supreme dilemmas. The Google DeepMind employee letter should encourage us all to ask questions. How is AI being used in this space? How are vendors ensuring their technologies are not misused? What safeguards are in place to prevent unintended consequences? Are there beneficial uses? Or are tech firms, and their desire to deploy at all costs, accelerating a global arms race and in danger of precipitating new conflicts?
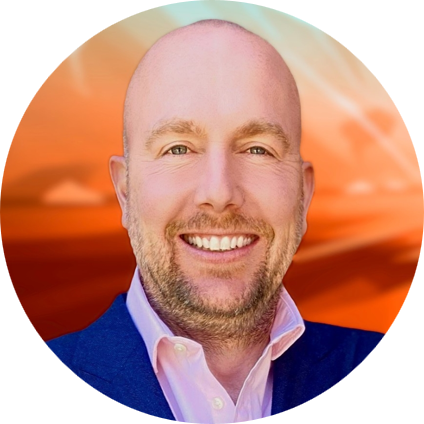
JOOST
Productivity, but not at any cost
The UK’s long-standing productivity woes may soon be a thing of the past, as artificial intelligence (AI) emerges as a potent solution. Recently, a Workday study revealed AI could potentially inject £119 billion annually into the UK economy by automating routine tasks and freeing up workers for higher-value activities. However, this productivity windfall comes with significant workforce disruptions, particularly in tech, apprenticeships, and gender equality.
AWS CEO Adam Selipsky’s statement this week that AI could soon take over much of the coding work traditionally done by developers has gained attention in the tech industry. This shift towards AI-driven programming threatens to redefine the role of software developers, potentially pushing them into supervisory positions over AI systems rather than hands-on coding, which will impact junior roles first.
And this extends beyond the tech sector, with traditional apprenticeship programs at risk of obsolescence. As AI automates entry-level tasks, young workers may struggle to find opportunities to gain practical experience, creating a skills gap that could have long-lasting effects on the workforce.
Gender disparities in AI adoption present another pressing concern. The World Economic Forum warns that women, who are overrepresented in sectors like administration and customer service, face a higher risk of job displacement due to AI automation. This trend threatens to exacerbate existing gender inequalities in the workplace.
Despite these challenges, the potential benefits of AI for UK productivity are meaningful. Business leaders could save up to 1,117 hours per year, while employees could reclaim 737 hours annually for more meaningful work. Industries such as finance, IT, and HR stand to gain significantly from AI-driven efficiencies.
The question now facing UK businesses and policymakers is how to harness AI’s productivity potential while mitigating its disruptive effects on the workforce. Can the UK strike a balance between innovation and job preservation? How can businesses ensure that the benefits of AI are distributed equitably across all sectors and demographics?
Takeaways: As AI reshapes the UK’s economic landscape, businesses must prioritise reskilling and upskilling initiatives to future-proof their workforce. Policymakers should consider targeted interventions to support sectors and demographics most at risk of AI-driven job displacement. Companies adopting AI technologies should focus on creating new roles that complement AI capabilities, rather than simply replacing human workers. By fostering a culture of continuous learning and adaptability, the UK can position itself to fully capitalise on AI’s productivity benefits while ensuring a smoother transition for its workforce.
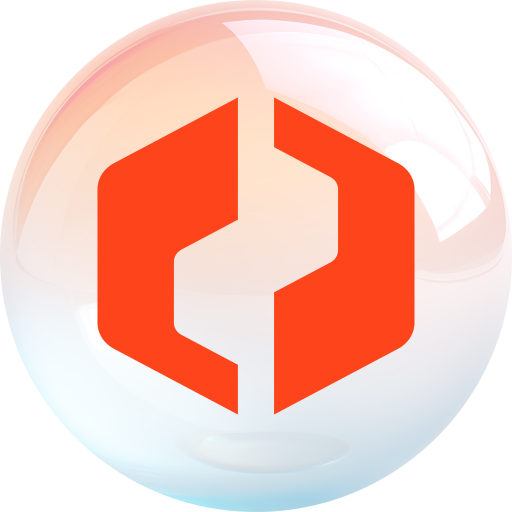
EXO
Weekly news roundup
This week’s news highlights the growing integration of AI in various industries, advancements in AI-generated content, ongoing debates in AI governance, and significant developments in AI research and hardware infrastructure.
AI business news
- Perplexity AI plans to start running search ads in fourth quarter (Indicates the monetisation strategies of AI-powered search engines, potentially challenging traditional search giants.)
- Ideogram 2.0 arrives, casting a shadow over Midjourney’s update (Highlights the rapid advancements and fierce competition in the AI image generation space.)
- An ‘AI scientist’ is inventing and running its own experiments (Demonstrates the potential of AI to accelerate scientific discovery, a theme we’ve explored in previous discussions on AI in research.)
- Zoom lifts revenue forecast on growing demand for AI tools used in hybrid work (Shows the increasing integration of AI in everyday business tools, supporting the trend of AI-enhanced productivity.)
- BeyondMath’s ‘digital wind tunnel’ puts a physics-based AI simulation to work on F1 cars (Illustrates the application of AI in high-performance engineering, showcasing its potential in specialised industries.)
AI governance news
- This system can sort real pictures from AI fakes — why aren’t platforms using it? (Addresses the ongoing challenge of distinguishing AI-generated content, a critical issue for maintaining trust in digital media.)
- California trims AI safety bill amid fears of tech exodus (Highlights the delicate balance between regulating AI and maintaining a competitive tech industry, a recurring theme in AI policy discussions.)
- Anthropic accused of violating authors’ copyrights (Continues the ongoing debate about copyright issues in AI training data, a topic we’ve covered extensively.)
- Did the FTC just ban AI-generated reviews? (Raises questions about the use of AI in consumer-facing content and its implications for trust and transparency.)
- Companies need more AI competency at board level, Norway’s wealth fund says (Emphasises the growing importance of AI literacy in corporate governance, a trend we’ve noted in previous discussions.)
AI research news
- Drama Engine: A framework for narrative agents (Explores the potential of AI in creative storytelling, an area of increasing interest for our readers.)
- Automated Design of Agentic Systems (Advances the field of AI agent design, potentially leading to more sophisticated and autonomous AI systems.)
- From pixels to planning: scale-free active inference (Presents a novel approach to AI perception and decision-making, bridging the gap between low-level sensory input and high-level planning.)
- Graph Retrieval-Augmented Generation: A survey (Provides an overview of an emerging technique that could enhance the capabilities of large language models.)
- LongWriter: Unleashing 10,000+ word generation from long context LLMs (Demonstrates significant progress in AI’s ability to generate coherent long-form content, a capability with wide-ranging implications.)
AI hardware news
- North America sees 70% jump in data center supply in construction, CBRE report says (Reflects the massive infrastructure expansion needed to support the growing demand for AI computing power.)
- SoftBank scraps AI chips tie-up plan with Intel, FT reports (Indicates shifts in strategic alliances within the AI chip industry, potentially impacting the competitive landscape.)
- Chinese imports of chip gear hit record $26 billion this year (Highlights the global race for semiconductor supremacy, a critical factor in AI hardware development.)
- NVLink and NVSwitch are Nvidia’s secret weapon in the AI wars (Explores the technical advantages that have contributed to Nvidia’s dominance in the AI hardware market.)
- These ‘living computers’ are made from human neurons — and you can rent one for $500 a month (Presents a cutting-edge development in biocomputing, blurring the lines between biological and artificial intelligence.)