Welcome to our weekly news post, a combination of thematic insights from the founders at ExoBrain, and a broader news roundup from our AI platform Exo…
Themes this week
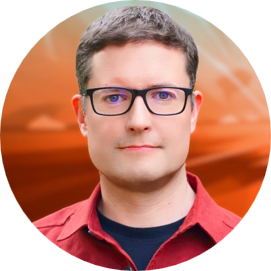
JOEL
This week we look at:
- Meta’s giant new Llama 3.1 405B open-weight model and its potential to reshape the landscape.
- Our co-authored white paper on the fund management industry’s AI-driven future.
- Google’s Olympic-level maths breakthrough and the practical roles AI will play at Paris 2024.
Llamas now graze on the open frontier
After much anticipation this week Meta unveiled the new Llama 3.1 ‘open-weight’ (freely downloadable) multilingual language model ‘herd’; this includes a distilled small 8B (8 billion parameter) model, a medium 70B variant, plus a new 405B monster.
Based on Meta’s benchmarks the 405B looks to be matching the most powerful ‘closed’ competitors such as GPT-4o and Claude 3.5 and leading the way in certain areas such as maths and scientific use-cases. This release really does mark a pivotal moment in AI development, as a model freely available for download now matches the capabilities of any cutting-edge, proprietary cloud hosted system. The implications are far-reaching, extending across business and the also the use by friendly and adversarial states, and as CEO Mark Zuckerberg puts it, this large model will provide a new option for training or distilling down into smaller specialised solutions.
405B is around 75% smaller (by parameter count) compared to the rumoured 1.8 trillion GPT-4 but demonstrate superior performance; this continues the trend of reductions in model size while improving capabilities, showcasing the advancements in training data cleansing techniques we’ve seen in the last year. Whilst the compute used to train likely exceeds any other model to date, the size reduction means reduced ongoing usage cost; ~$3 per million tokens, versus $6 and $7.5 for Anthropic and OpenAI’s best models respectively.
Downloading Llama 3.1 405B is one thing, but running this behemoth is another matter. The model tips the scales at around 810GB in its raw form, the rule of thumb for memory needs are 1.2x the size on disk. As Artificial Analysis pointed out, this means to run you’d need a system with more than the 640GB of high-bandwidth memory that comes with an industry standard 8x H100 GPU rig. So that means two Nvidia machines connected with high bandwidth interconnects, to get the 405B up and running… likely to set you back near £1 million (or $40k/month in the cloud)!
Enter quantization, the less talked about number that is increasingly important in AI deployment. Think of it as the MP3 bitrate of the AI world – a clever compression technique that allows us to squeeze more model into less memory. Just as MP3s made it possible to store thousands of songs on early music players, quantization enables us to run large AI models on more modest hardware. By reducing the ‘precision’ of the model’s parameters (it’s massive matrix of numbers) from 16-bit floating-point to 8-bit or even lower, it’s possible to slash memory requirements. Compress 405B to 8-bit and it will run happily on a typical 8 GPUs server. Thomas Scialom, the training lead on Llama 3.1 indicated on the Latent Space podcast this week that it’s the expectation the model will be run in it’s 8-bit form, and there is a real potential for this kind of compression to make AI more powerful at any given compute budget, including during training.
Quantization comes in several flavours, each offering a different balance of compression and accuracy, and both during and after training. The most straightforward method simply uses fewer bits to represent each number in the model, much like reducing the colour depth of an image. More sophisticated techniques include grouping similar values together or using variable levels of precision for different parts of the model. The quantization trade-offs are complicated. For many natural language processing tasks, the impact on performance is negligible. In these cases, a big model with high compression can run on much less memory and still beat a small model with no compression. However, for specialised applications like multilingual processing, coding, or complex mathematical reasoning the reduced precision can lead to very noticeable degradation in output quality. It’s a balancing act between model size, computational efficiency, and task-specific performance. 8-bit quantization in most cases sees very little reduction in performance, especially with larger models, which are more resilient to compression. 6-bit quantization is a good point of balance, but 4-bit and 2-bit generally see major negative impacts including in some cases, also slower throughput. Research we highlighted a few months ago proposes so-called “1-bit” quantization and Meta’s Scialom believes there is merit in the idea.
The availability of state-of-the-art open-weight models democratises access to cutting-edge AI capabilities to a degree. However, 400+B parameter models are not going to have a direct impact on the burgeoning world of running ‘small language models’ on your laptop just yet. Looking ahead, this is not going to be like the iPod era where the capacity of MP3 players grew fast while the costs dropped. The expensive high bandwidth memory used by these servers has reduced in cost recently but is expected to rise in the coming year (3-8% QoQ) given demand, high production complexity, and the introduction of the more expensive GDDR7. As memory capacity struggles to keep pace with model size, innovations in quantization and other compression techniques will become increasingly high-profile. Much effort will continue to shift to both getting more from fewer bits, and fundamentally new model architectures that can use memory more efficiently. The path to artificial general intelligence (AGI) is about both growing and compressing our newfound artificial brains.
Takeaways: Llama 3.1 405B represents a significant milestone in open-weight AI, but its sheer size limits its directly disruptive potential. Nevertheless, it levels the playing field and provides a powerful new tool to create new smaller models. Meta’s openness is also impressive, their technical report contains a huge amount of detail. With many service providers offering access already such a Groq, Together AI (try on their playground here), Amazon, and Google, it puts pressure on the likes of Anthropic and OpenAI to reduce their prices and offer more flexibility. Meta’s strategy is twofold: squeezing competitors, while warming up their 600,000+ GPUs to leverage their vast social training data (outside the EU at least). But while this release is a significant stepping stone towards more powerful AI, it also highlights some current barriers. Despite the ingenuity of model quantization, we’re still facing a memory cost wall that restricts the widespread deployment of large models. The race to overcome these barriers will accelerate, whilst Meta start training Llama 4. Next move OpenAI?
It’s do or die for asset management
As AI rapidly advances, its impact varies across industries. Despite ongoing technology adoption, the asset (fund) management sector is encountering a revenue and productivity plateau.
We’ve co-authored a white paper with our partners at fVenn and it examines how AI can revitalise asset management, exploring the industry’s unique position to harness the technology’s potential. We present a comprehensive transformation roadmap and argue that swift action is essential for firms to maintain their competitive edge in an evolving financial landscape.
Download the white paper here and read the companion article on CityWire.
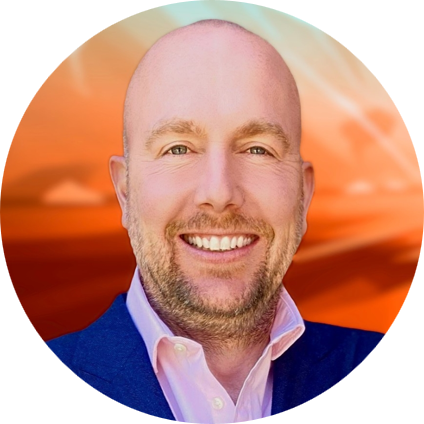
JOOST
AI goes for gold (and gets silver)
In last week’s newsletter, we explored the rapid advancements in AI’s mathematical capabilities. This week, that progress has been dramatically underscored as a Google DeepMind system achieved silver at the International Mathematical Olympiad (IMO). By solving four out of six problems, including the competition’s hardest question, the system demonstrated its ability to tackle challenges at a level comparable to the world’s brightest mathematicians. This achievement is particularly significant in terms of the mix of techniques used, combining LLMs, reinforcement learning, and formal mathematical proofs in a novel way. This synergy of different approaches demonstrates how complex problems can be tackled by leveraging inventive architectures. Its also interesting to see Gemini playing a key role in the solution, translating natural language problem statements into formal mathematical statements to help other AI components train themselves. More details will no doubt emerge, but this looks like another notable breakthrough by the DeepMind team.
Meanwhile AI’s influence isn’t confined to the realm of the abstract. As the sporting Olympiad gets underway in Paris today, AI is set to transform how we experience, participate in, and safeguard major sporting events. The International Olympic Committee (IOC) has identified over 180 potential use cases for AI in these games. Google is partnering with NBC and Team USA and will leverage Gemini to enhance viewer experience. Commentators will use AI-powered tools to provide quick insights into sports, athletes, and rules, making the games more accessible and engaging for audiences worldwide. NBC’s “chief superfan commentator,” Leslie Jones, will use Gemini to explore and explain new sports in real-time, adding a fresh dimension to Olympic coverage.
AI’s role in enhancing viewer experiences and providing personalised content could revolutionise how media companies engage with their audiences. NBCUniversal’s “Your Daily Olympic Recap,” which offers AI-generated summaries of favourite events narrated by an AI version of legendary sports presenter Al Michaels, points to a future where content is tailored to individual preferences at scale. AI is also pivotal in bolstering security around Paris. Systems have been deployed to monitor crowds and detect suspicious activities. This sophisticated surveillance system is designed to pre-emptively identify potential threats, providing an additional layer of security beyond traditional methods. Sports analysis is also in scope for enhancement: technologies like Intel’s 3D athlete tracking (3DAT) analyses biomechanics, potentially levelling the playing field by helping coaches identify talent and optimise training regimens.
Takeaways: AI’s Olympic prowess is diverse. Progress in maths competitions presages more powerful reasoning and scientific uses. Use in sporting events shows versatility but critics will rightly worry about privacy infringement and the normalisation of surveillance. This tension between security and civil liberties exemplifies the broader challenges of AI adoption. The Paris Olympics will no doubt keep us on the edge of our seats for the next two weeks, but it will also serve as a significant test case for the integration of AI across various aspects of a large global event.
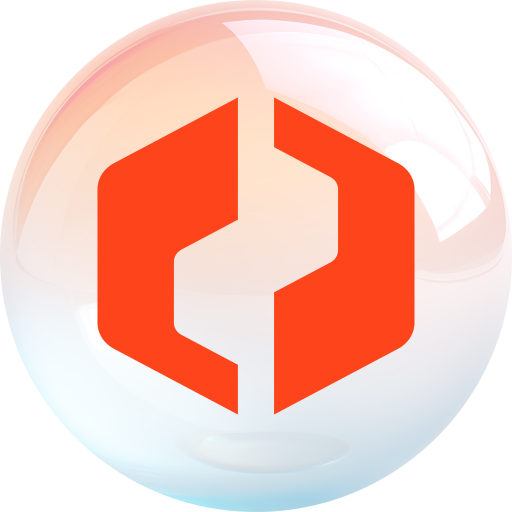
EXO
Weekly news roundup
This week’s news highlights the ongoing competition in AI model development, concerns over AI’s impact on various industries, continued investment in AI startups, and the race for AI hardware supremacy.
AI business news
- Mistral shocks with new open model Mistral Large 2, taking on Llama 3.1 (This development showcases the rapid progress in open-source AI models, potentially challenging the dominance of closed-source alternatives.)
- Hollywood’s videogame performers to strike over AI, pay concerns (Highlights the growing tension between AI technology and creative industries, echoing previous discussions about AI’s impact on various professions.)
- Tesla to have humanoid robots for internal use next year, Musk says (Demonstrates the potential for AI-powered robotics in manufacturing, a topic we’ve explored in relation to automation and job displacement.)
- VCs are still pouring billions into generative AI startups (Indicates continued investor confidence in AI’s potential, despite concerns about market saturation we’ve previously discussed.)
- OpenAI tests new search engine called SearchGPT amid AI arms race (Suggests a potential shift in the search engine landscape, which could have significant implications for how we access information online.)
AI governance news
- Anthropic does not support California AI bill SB 1047 (Highlights the ongoing debate between AI companies and regulators, a theme we’ve explored in discussions about AI governance.)
- TikTok Lite leaves up to 1 billion users with fewer AI protections (Raises concerns about AI ethics and the digital divide, particularly in developing countries.)
- Astronomers discover technique to spot AI fakes using galaxy-measurement tools (Demonstrates an innovative approach to combating AI-generated misinformation, a recurring theme in our discussions.)
- UK competition watchdog signs joint AI statement with US and EU (Indicates growing international cooperation on AI regulation, a crucial development for global AI governance.)
- What does Google Gemini do with your data? It’s complicated (Highlights ongoing concerns about data privacy and transparency in AI systems, a topic we’ve frequently addressed.)
AI research news
- Cooperative reward shaping for multi-agent pathfinding (Advances in multi-agent AI systems could have significant implications for logistics and urban planning.)
- OpenDevin: an open platform for AI software developers as generalist agents (This research could revolutionise software development, potentially leading to more efficient and accessible coding practices.)
- CoD, towards an interpretable medical agent using chain of diagnosis (Demonstrates progress in making AI medical diagnoses more transparent and understandable, addressing a key concern in AI healthcare applications.)
- A survey on employing large language models for text-to-SQL tasks (Highlights the potential for AI to make database querying more accessible, which could have wide-ranging implications for data analysis and business intelligence.)
- A survey of prompt engineering methods in large language models for different NLP tasks (Provides insights into the evolving field of prompt engineering, which is crucial for optimising AI performance across various applications.)
AI hardware news
- Training starts at Musk’s xAI GPU supercomputer facility (Demonstrates the massive computational power being deployed for AI training, a topic we’ve discussed in relation to AI’s environmental impact.)
- Exclusive: Nvidia preparing version of new flagship AI chip for Chinese market (Highlights the geopolitical complexities of the AI chip market, a recurring theme in our coverage of AI hardware.)
- Nvidia supplier SK Hynix posts 6-year high profit on AI boom (Illustrates the economic impact of the AI boom on the semiconductor industry, a trend we’ve been tracking.)
- Amazon racing to develop AI chips cheaper, faster than Nvidia’s, executives say (Indicates increasing competition in the AI chip market, which could lead to more affordable and efficient AI hardware.)
- Microsoft taps Lumen to bolster AI DC networks (Showcases the ongoing infrastructure investments needed to support the growing demand for AI computing power.)