Welcome to our weekly news post, a combination of thematic insights from the founders at ExoBrain, and a broader news roundup from our AI platform Exo…
Themes this week
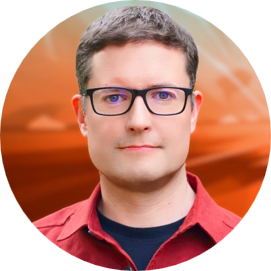
JOEL
This week we look at:
- Why the AI bubble narrative might be missing the bigger picture.
- A bold proposal to boost productivity in the public sector.
- How OpenAI are calibrating the path to artificial general intelligence (AGI).
Bursting the AI bubble narrative
This week, news sites and social media were abuzz with claims of Wall Street turning sceptical on artificial intelligence, citing recent analyses from Goldman Sachs, Sequoia Capital, and Barclays. As ever, a closer look reveals a different reality behind these sensationalised stories. These reports, far from representing a unified Wall Street perspective, stem from a handful of analysts whose views clearly don’t encompass the entire US financial sector, and certainly not those analysts who have even the most basic knowledge of AI’s development trajectory.
At the heart of their comments lies a basic argument: fear of missing out is driving big tech firms to ‘overbuild’ compute infrastructure creating a ‘bubble’. While it’s true that datacentre capex is above historical levels, and recent growth in cloud and AI business doesn’t yet show directly proportional revenue increases, the narrative of a bubble is an oversimplification. What seems to be happening here is that the comments of a few analysts are striking a chord with those who have yet to imagine what myriad uses powerful computational capacity can be put towards. This can be likened to IBM president Thomas J. Watson’s comments from the 1940s projecting a global market for “about five computers”. One can imagine there was a steam engine bubble, a railways bubble, an electricity bubble that formed in the minds of those still trying to grasp these general-purpose technologies, and perhaps confusing them with products and services.
Sequoia partner David Cahn’s analysis roughly estimates an annual gap of $600 billion between AI infrastructure spending and product revenue generation. This figure suggests some disconnect between investment and returns. Barclays analyst Ross Sandler draws parallels to the dot-com boom of the 2000s, where Internet bandwidth was deployed before business models for the Internet had been fully developed. However, this comparison overlooks crucial differences. Today’s tech giants, by contrast, enjoy robust financial positions and diverse businesses that provide a substantial cushion against slow take-off. The firms that collapsed during the dot-com era were in some cases spending more than 100% of their cash flow on building infrastructure (that in the end did prove highly valuable, albeit too late for those fragile businesses).
The concept of “over” building implies these analysts are able to predict future demand for AI capabilities and computational power – a notion their published comments fail to backup. Goldman Sachs’ Jim Covello, claims that AI isn’t designed to solve complex problems, citing experiences with “illegible and nonsensical results” in summarisation tasks. Such statements betray a surprisingly shallow understanding of AI’s current capabilities and potential. Barclay’s Sandler argues current AI capex will deliver compute in the next few years to power “12,000 new ChatGPT-scale AI products” – a capacity he deems excessive, again belying a limited understanding of the applications of intelligent compute that (as we have covered many times in this newsletter) go far beyond chatbots.
This narrow view stands in stark contrast to the perspectives of big tech leaders actively investing in the AI build-out. Amazon backed Anthropic’s CEO forecasts AI models costing $1 billion to train in the near term, with $100 billion models on the horizon. Microsoft’s CTO Kevin Scott continues to emphasise that he’s not yet seeing diminishing returns on AI scale-up, with each new generation of models bringing significant improvements in capability, cost-effectiveness, and robustness.
The impact of AI is already being felt across every conceivable sector, and manifests in digital systems, robotics, real-world and virtual-world simulations. While diffusion of these technologies isn’t immediate and adoption patterns are complex, dismissing AI’s potential on the basis of a few anecdotal experiences with the current generation chatbots and some back-of-a-napkin calculations is absurd. As with transformative technologies of the past – like steam, electricity, and internet bandwidth – the full scope of AI’s influence may take time to be effectively measured, but ‘artificial intelligence’ will be nothing other than intrinsically profound when it matures.
Takeaways: Businesses and investors should look beyond lazy headlines and limited analyst opinions when assessing AI’s potential. While caution on the scaling laws, energy and copper supplies, and the long-term effectiveness of current model architectures is warranted, dismissing the technology outright based on current revenue figures or isolated experiences with early applications will lead to missed opportunities. Instead, focus on understanding AI’s general-purpose capabilities, explore its novel use-cases and track its widespread adoption. Remember that new technologies often face scepticism in their early stages – opportunity awaits those who accelerate adoption.
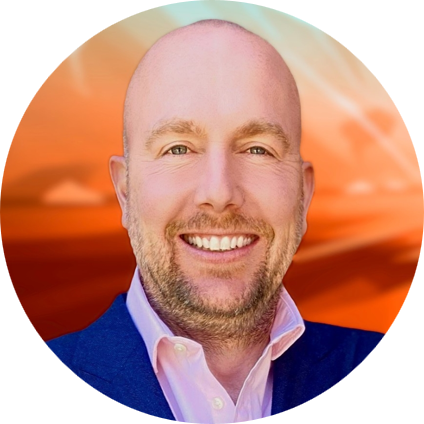
JOOST
Re-imagining public sector productivity
As Europe adjusts to post-election realities, reducing public sector spending and increasing productivity is a critical focus. The Tony Blair Institute (TBI) has issued a report at its conference this week; Governing in the age of AI: Reimagining the UK Department for Work and Pensions (DWP) outlining the kind of AI strategy governments may need to adopt in their most complex departments. The plan aims to leverage AI to streamline processes, potentially freeing up 40% of the workforce’s time and saving £1 billion annually.
The report suggests this level of productivity gain would have a profound effect on the cost, quality and volume of services the DWP provides to citizens, and would see it adopt three ‘signature policies’:
- Within a year, reduce backlogs for every type of benefit to zero to give every citizen the support they need when they need it.
- Reimagine job centres by introducing a digital employment assistant for every claimant so they can find the right job or training to progress in their career and gain financial independence.
- Turn the DWP into an “AI exemplar” department that spurs cross-governmental collaboration to drive economic growth and reduce the long-term cost of benefits.
While many countries are still in the early stages of AI adoption, often limited to service chatbots, the outlined strategy represents a somewhat more ambitious roadmap. The vision extends beyond cost-cutting, focusing on enhancing service delivery by reimagining job-related services. The potential for AI to drive efficiency in healthcare, the other giant to-do list item, also comes to mind. A recent World Economic Forum article highlighted AI’s role in reduce the cost of drugs, underscoring its potential transformational impact on public health.
However, the path to AI-driven public sector transformation is not without hurdles. Concerns about job displacement, data privacy, and the ethical use of AI in government decision-making must be addressed. The success of such initiatives will depend on careful implementation, robust safeguards, and public trust.
Takeaways: As AI continues to evolve, public and private sector leaders alike need strategies adapted to their own context. Besides having a plan and investing in AI literacy, the first, single most important step will be to start experimenting with AI, quicky realising benefits, and using those to drive further transformational change. The coming years will likely see a growing divide between governments that successfully harness AI’s potential and those that lag behind, making strategic AI adoption an increasingly critical factor in public sector performance and citizen satisfaction.
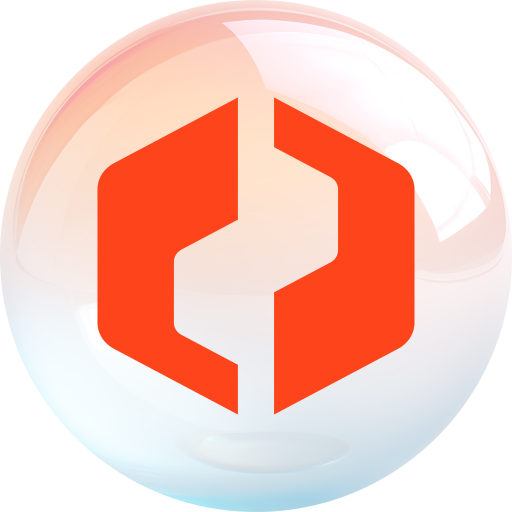
EXO
The age of reason
This week, OpenAI introduced a five-level system for tracking progress towards Artificial General Intelligence (AGI). The move comes as major AI labs increasingly talk of the next threshold of AI capabilities alongside safety considerations. OpenAI’s structure gives us a glimpse of the step-change new models might bring, and how we can think of future AI systems.
The new framework categorises AI into five levels: chatbots, reasoners, agents, innovators, and organisations. The company claims to be approaching Level 2, ‘reasoners’, which represents AI systems capable of human-level problem-solving equivalent to someone with a PhD-level education.
Level 3, ‘agents’, refers to AI systems that can work on tasks for several days on a user’s behalf. Level 4, ‘innovators’, describes AI capable of generating new innovations. The final level, ‘organisations’, suggests the ambition for AI systems that can perform the work of entire organisations. This roadmap hints at the potential capabilities of future AI models like GPT-5, and beyond that point, the potential for models to go beyond the boundaries of a single human equivalent to a more collective scale of intelligence.
While OpenAI’s scale focuses on capability, Anthropic uses a different approach. Their AI Safety Level (ASL) system, which ranges from ASL-1 to ASL-5+, emphasises safety measures and containment protocols required for models of increasing capability. Claude 3.5 Opus, Anthropic’s next frontier model, slated to come later this year, may well be pushing the boundaries of these classifications. If 3.5 Sonnet is anything to go by, its advanced reasoning abilities could be approaching ASL-3, which is characterised by “low-level autonomous capabilities”, as well as perhaps OpenAI’s level 2 ‘reasoners’ stage or beyond.
Takeaways: AI scales become more meaningful as we move through the gears. Businesses should view them as strategic tools for understanding the AI landscape and experimenting with future innovations in mind. These scales could also be enriched to support the kinds of financial projection we cover in this week’s newsletter, providing a more structured basis for understanding AI’s potential.
Weekly news roundup
This week’s news highlights the expanding integration of AI across various sectors, significant developments in AI hardware and research, and ongoing discussions about AI governance and ethics.
AI business news
- OpenAI startup fund and Ariana Huffington’s Thrive Global join to launch personalised AI health coach (This collaboration showcases the growing trend of AI applications in personal health and wellness, potentially revolutionising how individuals manage their well-being.)
- AWS introduces App Studio to speed up application development with AI (This development aligns with the ongoing trend of AI-assisted coding, potentially accelerating software development processes for businesses of all sizes.)
- Watch a robot navigate the Google DeepMind offices using Gemini (This demonstration highlights the practical applications of AI in robotics, showcasing how language models can be used for real-world navigation tasks.)
- With $6M in seed funding, Enso plans to bring AI agents to SMBs (This funding round indicates a growing interest in making AI technologies more accessible to smaller businesses, potentially levelling the playing field in various industries.)
- Xiaomi’s self-optimising autonomous AI factory will make 10M+ phones a year (This development represents a significant step in AI-driven manufacturing, potentially reshaping production processes and efficiency in the tech industry.)
AI governance news
- Chinese developers scramble as OpenAI blocks access in China (This situation highlights the geopolitical complexities surrounding AI technologies and their global accessibility.)
- Microsoft withdraws from OpenAI’s board (This move could signal shifts in the AI industry’s power dynamics and partnerships, potentially impacting future collaborations and developments.)
- Vimeo joins YouTube and TikTok in launching new AI content labels (This trend of labelling AI-generated content across major platforms reflects growing concerns about transparency and authenticity in digital media.)
- OpenAI breach is a reminder that AI companies are treasure troves for hackers (This incident underscores the critical importance of cybersecurity in AI companies, highlighting potential vulnerabilities in the industry.)
- Data workers detail exploitation by tech industry in DAIR report (This report sheds light on the often-overlooked human labour behind AI systems, raising important ethical questions about the AI industry’s practices.)
AI research news
- MInference 1.0: Accelerating pre-filling for long-context LLMs via dynamic sparse attention (This research could lead to more efficient processing of long texts in language models, potentially improving their performance and applicability.)
- FlashAttention-3: Fast and accurate attention with asynchrony and low-precision (This advancement in attention mechanisms could significantly enhance the speed and efficiency of AI models, particularly in resource-constrained environments.)
- PaliGemma: A versatile 3B vision model (This new vision model demonstrates progress in creating more flexible and adaptable AI systems for visual tasks.)
- Video-to-Audio Generation with Hidden Alignment (This research opens up new possibilities in AI-generated audio content, potentially revolutionising fields like dubbing and audio description.)
- Internet of Agents: Weaving a web of heterogeneous agents for collaborative intelligence (This concept could pave the way for more interconnected and collaborative AI systems, potentially leading to more sophisticated and adaptable AI solutions.)
AI hardware news
- Elon Musk reveals plans to make world’s “most powerful” 100,00 NVIDIA GPU AI cluster (This ambitious project could significantly boost AI computing power, potentially accelerating AI research and development across various fields.)
- AMD buys dev Silo AI in bid to match Nvidia’s AI portfolio (This acquisition signals intensifying competition in the AI hardware market, potentially leading to more diverse and advanced AI chip options.)
- China can lead in AI despite hardware restrictions, Huawei Cloud CEO (This statement reflects the ongoing global competition in AI development and the potential for innovation despite technological constraints.)
- AI frenzy takes Taiwan’s TSMC to record peak, puts it in trillion dollar club (This milestone underscores the growing economic impact of AI technologies on the semiconductor industry and global markets.)
- SoftBank acquires UK AI chipmaker Graphcore (This acquisition highlights the increasing value placed on specialised AI hardware companies and could lead to further advancements in AI chip technology.)