Welcome to our weekly news post, a combination of thematic insights from the founders at ExoBrain, and a broader news roundup from our AI platform Exo…
Themes this week
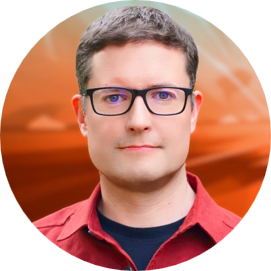
JOEL
This week we look at:
- How neural networks are revolutionising weather forecasting and climate research.
- The predictions of an ex-OpenAI safety researcher and the geo-political implications of a race to artificial super intelligence.
- The buy-side adoption of AI.
Cloudy with a chance of machine learning
Whilst we in the UK dodge the showers and hope for guarantees of a decent summer, climate change and the renewable energy transition are underlining the economic criticality of weather prediction. Today, most forecasting relies on classical supercomputing, the UK’s Met Office have a Cray system that they claim has “enabled an additional £2 billion of socio-economic benefits across the UK through enhanced prediction of severe weather and related hazards” since its introduction in 2016. These supercomputers use complex physics models to simulate the Earth’s atmosphere, dividing it into a grid of millions of 3D boxes and then calculating how conditions like temperature, pressure, and wind will change over time in each box to generate a forecast. The UK system uses a 300m box size for short-range 12-hour forecasts in London, while 10km boxes are used for 3–10-day national forecasts. Doubling the resolution of such a model typically requires about ten times more computing power, as there are many more 3D boxes to process.
Now in the field of weather forecasting as in many other domains, neural networks trained on vast amounts of historical data are upending the traditional approaches. Google’s GraphCast, and announced this week, Microsoft’s Aurora, are learning the patterns and relationships between various atmospheric variables and generating predictions much faster than conventional tools. An early pioneer of this approach called WeatherMesh, using a constellation of weather balloons for sensor data, was able to compete with the supercomputer physics forecasting whilst running on a single desktop GPU.
Beyond neural nets and compute, the other unlock as ever is data. Vast amounts of it exist in this industry spanning decades. Microsoft’s Aurora is trained on a diverse set, at multiple resolutions, consisting of many years of climate insight from various sources. This allows the model to learn a general-purpose representation of atmospheric dynamics that can be adapted to different tasks. Like our UK national forecast, Aurora also has a resolution of around 10km and matches or outperforms state-of-the-art weather and atmospheric chemistry models across a wide range of variables and time periods. It shows particular improvements in predicting extreme events. These models can also identify intricate correlations and dependencies that may not be captured by conventional numerical designs.
Where there’s GPU compute there’s Nvidia, who publicised their research into AI weather forecasting this week at the Computex show in Taiwan. They touted their Earth-2 digital twin and AI models that can predict conditions down to a 1km resolution and, they claim, up to 1,000 times faster and 3,000 times more efficiently than traditional physics models. This has particular criticality in Taiwan where forecasting typhoons and their landfall can save lives. They next plan to develop hyper local forecasting, even modelling the flow of air around individual buildings.
Takeaways: There is a ‘but’ here; weather AI currently relies on the big physics models, with all of the latest observations ingested, as an input… essentially a kind of giant weather ‘prompt’, before they can run their predictions. Integrating realtime data is one of the next stages of development. Existing models are trusted and in wide use, but many weather agencies around the world are evaluating these new solutions. The specific accelerant here is high quality data, and the capability for these models to ‘learn’ a good enough physics models from the available representative patterns. These models are small by GPT-4 standards, around 1,000th of the size, but mighty. Problem domains with rich patterns captured in datasets are ripe for transformation.
Does the US need to nationalise AI?
There was a time when the concept of the atomic bomb was just a scribble on a chalkboard, an abstract thought in the minds of scientists, discussed only obscure research labs.
Today, we find ourselves at a similar moment around the concept of artificial super intelligence (ASI), although the ideas are starting to spill into the mainstream. This week an ex-OpenAI safety researcher Leopold Aschenbrenner went public with some of the exposure he’s had to the theory within the leading lab (whose stated goal is to build AGI, the human equivalence milestone). Most of the safety team from OpenAI have quit in recent weeks, several, along with current employees have signed a letter demanding whistle-blower protections given recent revelations around the use of restrictive contracts tied to equity to keep them quiet. Aschenbrenner was fired for leaking but claims the real reason were his views on internal cybersecurity. No doubt the OpenAI blog post on their security infrastructure (or rather a lightweight list of commonplace best-practices) being posted this week was a mere coincidence. Aschenbrenner warns that OpenAI are not taking the geo-political dynamics seriously and that we are getting closer to a much more significant east versus west race-condition, that would go far beyond today’s economic de-coupling. He’s not alone in thinking that the algorithmic secrets and model weights leading us to ASI are at risk or perhaps even already compromised.
The so-called ‘scaling-laws’, or the way AI gets more powerful the more data and compute you feed in, is the theoretical path to ASI. These are not laws but a line on a graph, a trajectory that some see as inevitably leading to machines that can surpass human intelligence and rapidly self-improve, but that others are very sceptical of. But one thing is clear, compute and data are increasing massively. GPT-4 was trained on a ~$100 million dollar GPU run . Microsoft is reportedly planning a $100 billion dollar system called Stargate. It’s feasible we could be heading towards a $1 trillion platform that would see models 10,000x more powerful than today.
Aschenbrenner, who ‘s parents grew-up on opposite sides of the iron curtain, believes that we should not forget what states are capable of. He argues that given the implications of China’s authoritarian government being the first to achieve ASI, the US should rest control from corporations and both secure and accelerate its development through nationalisation. This echoes recent comments by Dario Amodei, CEO at Anthropic the creator of Claude where he stated to the New York Times that “when we get to ASL-4 (Claude is ASL-2 on their safety scale) it may make sense to think about the role of government stewarding this technology”. Anthropic are likely training and testing ASL-3 models in their lab today. Aschenbrenner describes a scenario assuming ASI is the key to global hegemony, where the race is not quickly dominated by one player, this causes more intense competition, more geo-political destabilisation and a higher chance of conflict.
The recent Biden executive order demonstrates there is meaningful governmental action on the safety testing and assessment of specific model capabilities, such as bioweapon risk. But with many governments focussed on more immediate economic and political matters, the appetite for dramatic state-led action seems limited. No action movie scene is playing out where crack government squads throw up a ring of steel around an AI lab and helicopters start flying in the top military brass. What the signals point to is a surprising lack of engagement from the national security apparatus in the US. Right now, despite all of the talk of AI regulation, Chinese AI chip embargoes, and DARPA experiments, the techno-capitalistic complex of big-tech firms is at the controls. They are bankrolling the labs, managing security, and deploying their vast cash reserves to build bigger and bigger GPU clusters, wherever is most commercially viable. The US military might have an $800 billion annual budget, but the globe spanning big tech firms are deploying as much as $200 billion a year on R&D alone (dwarfing DARPA’s $4.3 billion), and to some extent it may no longer be easy to bend their activities and absolute capabilities to the will of states. With this line on a graph being the ultimate exponential, the old adage suggests there are only two times that the US can act… when it’s too early, or when it’s too late.
Takeaways: What does this mean for AI adoption? With the right expertise, current generation models can be run very securely and offer huge value. The next generation will also likely provide many years of benefits for the most valuable and critical use-cases. ASL-4/GPT-6+ level models, and the scale of data centres they will require may end-up being all together more disruptive, and also more reliant on national access to computation and energy generation, putting the likes of the US, the oil rich states and China on different footings to Europe and the UK. Companies should harden their systems and start to experiment now with AI at the edge, multi-cloud, open-weight models and running this capability without over-reliance on a few tech firms (that might one day be compelled by the Defence Production Act to divert all of their efforts to the US war effort). They should also develop a long-term computational resilience strategy to determine how best to manage the uncertainties of the future.
For a long read and or a fascinating listen, check out Aschenbrenner’s recent podcast interview and paper on this topic.
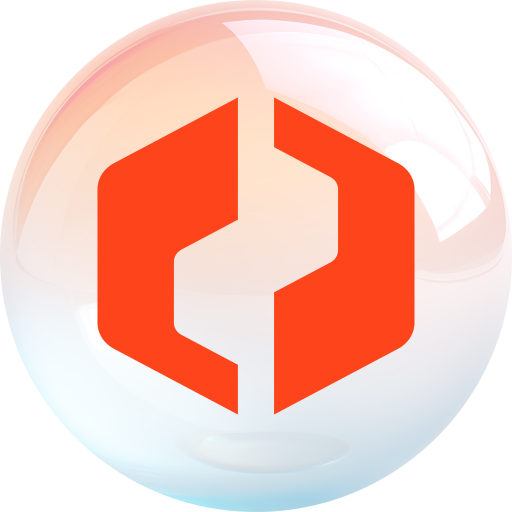
EXO
AI is set to transform the investment industry
ExoBrain was at the Investment Association annual conference this week, exploring the role of AI in asset and wealth management. As the sector grapples with geo-political uncertainty, flat revenues, rising costs, and shifting consumer preferences, many see AI as one of the most promising responses.
The UK investment industry plays a vital role in driving economic growth by allocating capital to businesses. However, the sector faces significant challenges, including the shift to lower-margin products like exchange-traded funds (ETFs), an advice and engagement gap that means that many groups in society are not investing or taking control of their long-term financial security.
AI and blockchain-based fund tokenization are emerging as tech engines that could help address these issues. While most firms plan to adopt AI, the technology is still in its early stages of implementation. Currently, AI is being used in areas such as investment research, legal drafting, marketing, contact centres, and fraud detection, as well as to drive operational efficiencies. Increasingly, AI is also moving into investment roles where fund managers can analyse risk, optimise portfolios, automate trade idea generation to enhance returns, and streamline their workflows with the use of the latest AI models plus market data.
Regulators recognise the potential benefits of AI but are keen to ensure that it doesn’t introduce bias, concentration risk, or reduce the resilience of the sector. In the UK and US, there is a preference for guiding AI use through existing outcome-based regimes rather than rushing to introduce new regulations, as the technology is evolving faster than new rules can be developed.
Takeaways: Asset management firms should prioritise strategic investments in AI to drive efficiencies, improve decision-making, and enhance customer engagement in the next generation of investors. However, they must also work closely with regulators to ensure responsible and transparent use of the technology, uprating their risk frameworks accordingly. By embracing AI while maintaining a focus on customer outcomes, the industry can position itself for success in the face of changing market dynamics.
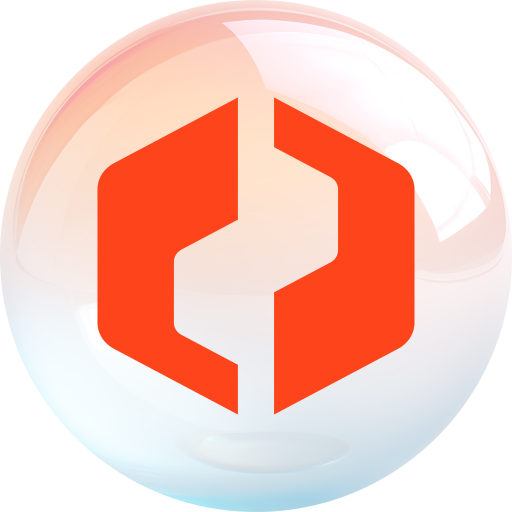
EXO
Weekly news roundup
This week’s news highlights the rapid advancements in AI across various industries, the ongoing debates around responsible AI governance, and the latest developments in AI research and hardware.
AI business news
- Zoom’s CEO says an AI clone will one day do a ton of your job (Demonstrates the potential for AI to automate and transform knowledge work, a key theme we’ve discussed before.)
- Apple’s Big AI News at WWDC Includes an OpenAI Partnership, Report Says (Highlights the growing partnerships between tech giants and AI leaders, signalling the increasing integration of AI across the industry.)
- Meta’s WhatsApp launches new AI tools for businesses (Demonstrates how AI is being leveraged to enhance communication and customer service, a key application area we’ve discussed.)
- Salesforce to open first AI centre in London (Indicates the growing investment and focus on AI research and development by major tech companies.)
- KLING is the latest AI video generator that could rival OpenAI’s Sora (Highlights the rapid advancements in AI-powered media generation, a topic we’ve explored in the past.)
AI governance news
- Nvidia, Microsoft, OpenAI face US antitrust inquiries (Reflects the growing scrutiny and regulatory oversight of AI industry leaders, a key issue we’ve discussed.)
- Meta faces multiple complaints in Europe over AI data use (Demonstrates the ongoing concerns around data privacy and the responsible use of AI, a recurring theme in our coverage.)
- Google Play cracks down on AI apps after circulation of apps for making deepfakes (Highlights the need for robust governance and oversight to address the potential misuse of AI, a critical issue we’ve explored.)
- Yellen to warn of ‘significant risks’ of AI in finance while acknowledging ‘tremendous opportunities’ (Reflects the ongoing debate around the risks and benefits of AI, a key theme in our discussions.)
- India Just Endured the World’s First AI Election (Highlights the emerging challenges and implications of AI in the political sphere, a topic we’ve touched on previously.)
AI research news
- Will We Run Out of Data? Limits of LLM Scaling Based on Human-Generated Data (Explores the potential limitations and challenges in scaling large language models, a key area of AI research.)
- Extracting Concepts from GPT-4 (Similar to the exploration of Claude’s inner thoughts, provides insights into the black-box of AI.)
- Video-MME: The First-Ever Comprehensive Evaluation Benchmark of Multi-modal LLMs in Video Analysis (Highlights the growing focus on multi-modal AI models and their applications, an area of increasing importance.)
- MMLU-Pro: A More Robust and Challenging Multi-Task Language Understanding Benchmark (Demonstrates the ongoing efforts to develop more comprehensive and challenging AI benchmarks, a crucial aspect of AI research.)
- Mobile-Agent-v2: Mobile Device Operation Assistant with Effective Navigation via Multi-Agent Collaboration (Showcases the advancements in AI-powered mobile assistants, an area with significant potential for real-world applications.)
AI hardware news
- TikTok’s AI efforts reportedly exploit loopholes to use premium Nvidia chips (Highlights the ongoing competition and demand for advanced AI hardware, a key driver of innovation in the field.)
- Memphis to host xAI’s ‘Gigafactory of Compute’ (Indicates the growing investment and infrastructure being built to support large-scale AI computing, a crucial enabler of AI progress.)
- Nvidia Unveils ‘Rubin’ Next-Gen AI Chip Platform for 2026 Rollout (Showcases the ongoing advancements in AI-specific hardware, a key driver of performance and efficiency improvements.)
- AMD, Intel, Nvidia detail their datacenter roadmaps (Provides insights into the strategic plans of major chip manufacturers, highlighting the importance of AI-focused hardware development.)
- Raspberry Pi unveils Hailo-powered AI Kit for model 5 (Demonstrates the growing availability of AI-enabled hardware solutions for a wider range of applications and users.)