Welcome to our weekly news post, a combination of thematic insights from the founders at ExoBrain, and a broader news roundup from our AI platform Exo…
Themes this week
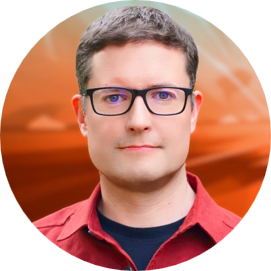
JOEL
This week we look at:
- The latest approaches being taken to standardise AI testing, with new assessments published by Scale AI.
- A new super-fast realtime speech synthesiser leveraging the new ‘state space’ model architecture, from Cartesia.
- Google’s issues with pizza glue, rock eating, and space cats as its AI summarised search results roll-out.
Testing AI
As the UK’s year 11 and 13 pupils grapple with the joys of standardised testing, the world of AI finds itself in a similarly painful state. While GCSEs and A-levels are rationalised as vital for comparing student achievement and school performance over time, many argue that these tests fail to reflect students’ true abilities and narrow the focus of teaching to just the tested subjects and content.
As more models become available, the question arises: how do we determine which are best, and are labs pouring too much effort into beating each other on standard tests rather than developing well-rounded capabilities? Are tests akin to human examinations the best approach?
This week Scale AI, the controversial AI data unicorn, has launched the SEAL Leaderboards to address the lack of transparency around AI performance. Their rankings use private, curated datasets and keep evaluation prompts under wraps to prevent labs from cheating. While this is progress, Scale AI only plans to update SEAL a few times a year and they cover a limited model subset. The methodology they have published is worth reviewing as a pretty comprehensive ‘test & evaluation’ approach for AI.
MMLU, or Massively Multi-Task Language Understanding, has been the focal benchmark for AI to date. It tests accuracy across 15,000+ questions spanning maths, science, history, and various problem-solving challenges. LLMs now regularly top 90%, beating out human experts in each field. However, many have highlighted the poor quality of the question set, and the likelihood that models are now highly tuned to perform well.
The LMSYS Chatbot Arena, a crowdsourced platform where humans ‘blind taste’ AI responses, has collected over 1,000,000 human comparisons to rank LLMs on a chess-style Elo scale. However, OpenAI has recently been accused of ‘style-hacking’ LLMSYS by formatting outputs with bullets and headings to make them look superficially better, even if the substance is inferior.
Many new tests like MMLU-Pro, with a better question set, and MMMU, which tests image recognition and multi-modal capabilities, have emerged. And then there are also questions of hallucination (HHEM), plus speed, and cost (analysed in detail by Artificial Analysis) to consider.
Like GCSEs, standardised tests have their place, providing a way to approximately compare groups of models against common tasks, and identify the ‘classes’ of capability. However, just as we don’t rely solely on a person’s GCSE results when hiring them for a job, nothing will beat assessing a model in context and judging it on its merits for a given role.
Takeaways: We’ve put together the latest report card across the most widely tested models; use it to get a feel for approximate strengths and weaknesses before testing your model in situ:
- Despite its troubles with the launch of AI in search, Google’s post I/O model updates are going much better and have seen the Gemini 1.5s surge up the table.
- GPT-4o is an all-new model, and heavily tuned to be capable and fast, and it performs well across the board. But more than any other model, given its so new, the scores should not be taken at face value when evaluating for a use-case. One area where it is clearly deserves it grades is multi-modal performance; it is a step forward in visual understanding.
- Good old GPT-4 Turbo tops the new SEAL coding table, born out by many comments on 4o’s coding skills, but suffers in the cost and performance stakes.
- Claude 3 has been slipping back. Some suggest its falling victim to the unexplained decay that many models seem to go through, often due to being victims of their own success, requiring behind the scenes performance optimisation that rob them of some of their initial zing. It’s also not showing well in the hallucination stakes.
- Llama 3 and that big Meta quality investment continues to impress, with the 70B model competing with its closed-source peers.
Realtime state-space speech
State-space architectures (SSMs) are a notable alternative to the all-conquering transformer design of ChatGPT, Gemini and Claude. An interesting use-case for more efficient SSMs emerged this week. Cartesia who have been pioneering in the area, released Sonic, a new voice model for high-quality lifelike audio. It has a latency of just 135ms, making it the fastest in its class. Essentially it can generate speech from text in a range of voices almost instantaneously, great for user interactions and voice powered solutions.
It also claims to be able to clone a voice with less than a minute of audio. Our initial experiments found the pre-prepared voices much more reliable than the cloned ones, but the potential is huge.
At ExoBrain we’ve recently been experimenting with ElevenLabs. It’s AI voice generator converts text into natural-sounding speech in 29 languages. It supports various accents and styles and is the most common AI TTS (text-to-speech) solution in use today. ElevenLabs targets content creators, writers, game developers, and businesses looking to create audio experiences, but lacks the near-instant generation of Cartesia.
You can experience voice generation in a new podcast version of this newsletter. Listen to last week’s episode here on Spotify which showcases a range of ElevenLabs voices, and this week using a cloned voice workflow embedded below. Whilst they sound pretty good, one of the issues with AI synthesis is its monotonic nature, especially if there is a single narrator…
Takeaways: We’re using a new workflow, based on a full voice cloning which improves the natural feel. If you want to quickly generate audio material the following setup and workflow is worth exploring:
Setup:
- Signup for ElevenLabs and clone your voice. You’ll need to verify its you by reading out a generated phrase; being allowed to clone somebody else’s voices would be highly problematic.
- We’ve found that a good quality mic and recording under a strategically draped duvet to deaden the sound helps to improve the capture. You’ll need to record a minimum of 10 minutes of training data, but more is better.
Workflow:
- Claude or Gemini do a great job of writing scripts from source text. Tell them it’s a podcast and they are able to structure in a suitable way, but some editing will be required.
- ElevenLabs ‘projects’ allow a large amount of text to be converted to speech, but the monotony will creep in to the resulting audio. We convert the AI script into our cloned voice first, section by section, which speeds narration up and introduces more variation.
- We then use the voice-to-voice generation tool, feeding back in the cloned audio to generate more varied output with one of the many professional voices on offer.
- Finally, we cut together in an audio editor (using AI music and effects from Suno).
For now, the state of the art is quite impressive, if not yet entirely convincing. The use of fast new architectures, and OpenAI’s yet to be released Voice Engine hints and significant progress to come.
Google’s search troubles
2-weeks ago we covered the announcements around Google’s much vaunted AI search results. Since then, things haven’t been going so well, with numerous examples popping up of absurd answers such as suggesting putting glue on pizza (based on an old Reddit post), claiming that cats have been found on the moon, and stating that most doctors recommend eating rocks.
Yesterday Google published a detailed response and action plan. They claim the issues are due to misinterpreting the original queries, struggling with ‘satirical’ material, content voids, and over-reliance on user-generated output. Unlike us humans, perhaps the AI is yet to appreciate the highly ‘variable’ nature of the things we find online. In response, Google is implementing a series of improvements, including better detection of nonsensical queries, limiting the use of user-generated content, enhancing protections for sensitive topics like news and health, and making enhancements based on the latest identified patterns. But the concern many highlight is that its likely the more subtle errors, not picked up by these changes, that will have a bigger impact.
Google believes ‘AI Overviews’ are needed and beneficial as they provide quicker summaries that directly answer user queries. They are designed to “take the work out of searching by providing an AI-generated snapshot with key information” and helping users get collated answers when the information they are looking for comes from multiple sources. Google claim that their research shows people prefer these results. Of course, the other big driver here is that Google feel they need to keep pace with competitors such as Perplexity who are re-building the search experience around AI.
Perplexity’s new Pages feature announced this week takes a different approach – rather than brief summaries, it generates an entire customisable webpage based on prompts. The goal is more comprehensive research reports, building on the AI powered search engines approach of combining referenced sources with generated analysis. Pages makes this research more sharable, but the nature of AI generation still requires the user to read, and sense check the output, and often to review a few of the cited sources to ensure the model has got the right gist. This is a huge time saver for research, but not a panacea.
Takeaways: Google is likely to take things more slowly, but the processing of extensive and diverse web search results is still a killer feature for AI. Perplexity look to be leading on how this is going to work in practice. Whilst Google will need to rebuild trust in its AI search features, for us users, the reality is that we should never trust anything digital on first contact. As the old (1993) joke goes… on the Internet nobody knows you’re a dog… or perhaps a spacefaring cat.
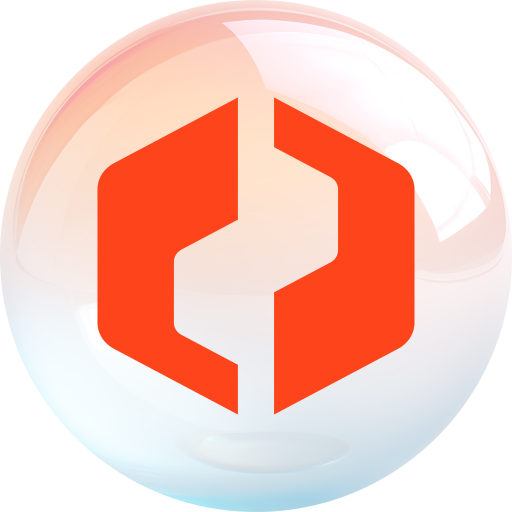
EXO
Weekly news roundup
This week’s news highlights the rapid advancements in AI across various industries, the ongoing debates around AI governance and regulation, exciting breakthroughs in AI research, and the critical importance of AI hardware development.
AI business news
- How Canva is approaching B2B customers with AI-powered tools (Showcases how AI is transforming the design and marketing software industry, a key focus area for ExoBrain.)
- PwC becomes first reseller of ChatGPT Enterprise in US and UK (Highlights the growing enterprise adoption of large language models and the opportunities for professional services firms.)
- Elon Musk’s xAI raises $6B from Valor, a16z and Sequoia (Demonstrates the continued investor interest in AI startups and Elon Musk’s ambitious plans in the AI space.)
- Microsoft reportedly unhappy about Apple’s OpenAI iPhone (Sheds light on the competitive dynamics in the AI platform and ecosystem space.)
- AI tutors are quietly changing how kids in the US study, and the leading apps are from China (Highlights the global race for AI-powered education solutions and the potential implications for the US market.)
AI governance news
- OpenAI researcher who resigned over safety concerns joins Anthropic (Demonstrates the ongoing debates around AI safety and the efforts to address these concerns.)
- AI and Covert influence operations: Latest trends (OpenAI explore how bad actors are using its tech)
- Former OpenAI board member Helen Toner reveals why Sam Altman was fired in bombshell interview—’we learned about ChatGPT on Twitter’ (Provides insights into the internal dynamics and governance challenges at leading AI research organizations.)
- With the EU AI Act incoming this summer, the bloc lays out its plan for AI governance (Examines the evolving regulatory landscape for AI and the potential implications for businesses and researchers.)
- California Gov Newsom warns against over-regulating AI (Highlights the ongoing debates around the balance between AI innovation and responsible governance.)
AI research news
- GPT-4 Better Than Humans At Financial Statement Analysis: Study (Demonstrates the impressive capabilities of large language models in specialized domains, a key area of interest for ExoBrain.)
- Transformers Can Do Arithmetic with the Right Embeddings (Showcases the ongoing advancements in AI research and the potential for transformers to handle complex tasks.)
- AI Starts to Sift Through String Theory’s Near-Endless Possibilities (Highlights the application of AI in fundamental scientific research, an area of growing interest for ExoBrain.)
- Faithful Logical Reasoning via Symbolic Chain-of-Thought (Showcases the ongoing research efforts to improve the reasoning capabilities of AI systems.)
- LLMs achieve adult human performance on higher-order theory of mind tasks (Demonstrates the advancements in AI’s ability to understand and reason about complex social and cognitive phenomena.)
AI hardware news
- Samsung workers’ union announces first-ever strike for chipmaker (Highlights the challenges and labor issues in the critical semiconductor industry, which underpins the development of advanced AI hardware.)
- Tech giants form an industry group to help develop next-gen AI chip components (Showcases the industry’s efforts to diversify the AI hardware ecosystem and reduce reliance on dominant players.)
- China’s $47B semiconductor fund puts chip sovereignty front and center (Highlights the global competition and strategic importance of semiconductor technology, which is crucial for AI development.)
- AI chip sales predicted to jump by a third – then cool off (Provides insights into the market dynamics and growth projections for AI hardware, a key area of focus for ExoBrain.)
- Elon Musk reportedly building ‘Gigafactory of Compute’ for AI (Highlights Elon Musk’s ambitious plans to develop specialized AI hardware, which could have significant implications for the industry.)