Welcome to our weekly news post, a combination of thematic insights from the founders at ExoBrain, and a broader news roundup from our AI platform Exo…
Themes this week
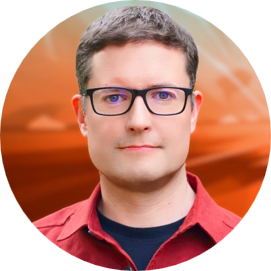
JOEL
This week we look at AI in healthcare, Lama 3, and LLM linguistics:
- A new study reveals GPT-4’s potential in ophthalmology, we look at AI powered personalised medicine
- Meta and Mark Zuckerberg mean business with their next-gen model
- We ‘delve’ in and explore the tell-tale signs of AI-generated text
The global healthcare crisis
Global healthcare is in a serious predicament: spiralling budgets, not enough workers, too many patients.
This is only set to worsen with aging and growing populations, and a stark global divide in care access, where low and middle-income countries annual per capita health expenditure is 60x lower than the likes of the US.
A Cambridge University study published this week added to the growing evidence that AI is demonstrating meaningful capabilities across many healthcare uses. In a series of tests GPT-4 went head-to-head with ophthalmologists at various stages of their careers, including unspecialized junior doctors, trainee eye doctors, and experts. The results were striking. GPT-4 significantly outperformed unspecialized junior doctors, whose level of specialist eye knowledge is comparable to that of general practitioners. There are now hundreds of research and real-world examples of large pre-trained and specialised models working well across various domains, including diagnostics, drug discovery, targeted medicine, and administrative processes.
As far back as the 1960s Harvard Medical School built the first computer capable of asking patients about their medical history, but in the intervening years the industrialised ‘one size fits all’ approach to healthcare has remained dominant. Now the rapid advancements in AI and genomics offers a new path, a transformative opportunity to reboot healthcare and bring in an era of personalisation universal access. By leveraging data, AIs can identify patterns and variations linked to specific diseases and responses, enabling targeted treatments that are more effective, and crucially more cost-efficient, using far less in the way of expensive drugs. AI can power predictive modelling to identify disease risk, speed up drug development and repurposing, aid diagnostics, automate genomics and pharmacogenomic analysis, assess remote monitoring via wearable tech, enable disease subtyping for new targeted therapies, and assist decision-making for treatment plans. This more personalised medicine is a model that focuses on quality rather than volume. Some are still sceptical, and the public aren’t yet sold, but such views don’t consider the realities; without a revolution healthcare we’re in trouble.
AI is making particular strides in personalised oncology. AI-driven genomic analysis is revolutionising cancer care by identifying the specific genetic mutations driving a patient’s illness. Companies like PathAI and Paige are analysing pathology images and finding specific genetic markers in cancer cells. This enables more precise diagnoses and targeted treatment plans. In the field of medical imaging, startups like Rad AI are using AI to improve the accuracy and efficiency of screening and diagnosis. Cancer is the second leading cause of death globally, and with about 70% of deaths from cancer occurring in low- and middle-income countries, personalised AI driven solutions would have a huge impact on global mortality.
Access to healthcare is a problem for us all, even in the UK. Many illnesses go undiagnosed, costing far more to the individuals and the NHS budget in the long run. Companies like Livv are developing consumer-facing AI applications that can help patients better understand their health data and make informed decisions about their care. Nvidia and Hippocratic AI’s recent partnership is creating AI nurses, offering highly tailored medical advice for just $9 per hour. That’s 4x less than the human nurse average and will drop as other efficiencies come onstream. And even surgery is seeing the potential for robotics to expand access, with more than 12 million procedures so far been performed by Intuitive’s robotic systems. For low and middle-income countries, AI-driven personalized medicine offers a promising path to leapfrog traditional healthcare barriers and provide more accessible, cost-effective, to their populations.
Data privacy and security are of-course a concern but companies like Unlearn and Syntegra are working to address these challenges by developing secure, anonymized clinical datasets.
The future of reliable widespread healthcare lies in the convergence of computational life sciences and AI, delivered through personalized medicine. As our global compute resources grow exponentially, we should urge governments and institutions to ringfence a meaningful proportion of that processing budget to make widespread and truly personalised medicine a reality.
Takeaways: Various NHS + AI trials are ongoing in the UK, many of which can be found on the NIHR’s website, and various UK firms are blazing a trial for AI services such as Huma, Cera and Healthily. (with their smart symptom checker).
Llama 3 unveiled
As anticipated Meta just unveiled Llama 3, its next generation of its state-of-the-art open models. The release this week includes 8 billion and 70 billion parameter versions, with a more powerful 405 billion model still in training (expected to be out in the Summer). Llama 3 demonstrates leading performance on industry benchmarks, with the largest expected to exceed GPT-4 levels and brings to the table new capabilities such as improved reasoning achieved with a much bigger training corpus than Llama 2.
The impact of Llama 3 is not limited to research labs and developer communities. Meta AI, the company’s flagship AI assistant, has been upgraded with v3 under the hood, elevating it to become a highly capable and prominent presence across Meta’s huge ecosystem of apps. Users can now enjoy cutting-edge features like real-time image generation, animations, and integration with Google and Bing search. Mark Zuckerberg, Meta’s CEO, asserts that Meta AI, powered by Llama 3, is now the most intelligent and freely available AI assistant on the market.
In a podcast interview with Dwarkesh Patel, Zuckerberg shed light on Meta’s ambitious efforts to achieve artificial general intelligence (AGI) and the company’s substantial investments in AI compute infrastructure (over 600,000 AI chips and counting). He emphasised that while narrow AI assistants were initially seen as sufficient for Meta’s products like WhatsApp and Instagram, the company came to realize that AGI-level capabilities would be essential for their systems to truly engage users in natural, and sophisticated interactions. Zuckerberg stressed the importance of imbuing AI with strong reasoning abilities, meaning Llama 3 has been trained on code, where Llama 2 was not. Whilst people likely won’t be generating C++ code on Facebook, this structure logical data provides high quality training for strong general reasoning skills.
Meta’s ‘open weights’ release of Llama 3 is part of a broader strategy to promote a balanced and responsible AI ecosystem. Zuckerberg states that concentrated AI power in the wrong hands poses a major risk, and Meta aims to avoid a closed, gatekeeper model. While the company will carefully evaluate each release to ensure safety, the goal is to make these powerful AIs accessible to a wide range of developers and businesses.
Takeaways: Most of the exciting open-weight models released in recent weeks have now been blown out of the water by Llama 3. ExoBrain’s initial testing suggests the small 8b model will be hugely capable for its size. We’ll see how the performance stacks up, but Meta mean business. Meta trained Llama 3 on much more data per model size than anyone else has previously used, and by some estimates 2x the compute was invested into the big 400b model than GPT-4! It sounds like they have really worked on training data quality too. But Meta only used about 1/10th (48,000 chips) of their amassed compute. All of this points to the huge future potential still to be deployed, and assuming scaling holds, Meta is gearing up to deliver AGI in the next few years and making everything available to the businesses and to system builders along the way. The Meta AI assistant is still only available in the US, but will be a great option for quick, simple AI tasks including rapid image generation. You can also try these new models on the Together.ai Playground.
‘AI-ese’ and the detection-stealth arms race
You’ve probably experienced that slightly odd feeling you get when reading AI generated text, even when it’s grammatically and factually correct. That sense that something is just a little ‘repetitive’. As it turns out, one of the most distinctive tells of ChatGPT’s language in particular, is its curiously frequent use of the word “delve”.
Researcher Jeremy Nguyen found that around 0.5% of medical research articles on PubMed now contain ‘delve’, 10 to 100 times more than just a few years ago, indicating both the increasing use of the technology to generate content, and this strange linguistic phenomenon. This week a Guardian investigation looked into this and proposed an explanation.
Whilst AI models are trained on a giant mass of text data (typically trillions of words or ‘tokens’), the transformation into an assistant requires human workers to test the system, provide feedback, and even write ideal responses. However, such a labour-intensive process has a crucial drawback, cost. To make such a system economically viable, the big AI firms outsource this essential human feedback (RLHF) work to lower-cost labour markets, such as those in Africa. These workers may have unconsciously imbued ChatGPT with elements of African business English, in which words like ‘delve’, ‘explore’, ‘tapestry’, and ‘leverage’ are more commonly used compared to American or British usage.
The distinctive voice of GPT-3.5 and 4 is a testament to the hard work and linguistic diversity of its human trainers, and their role should get more attention. The use of low-cost labour to train AI models is a troubling example of how the drive for ever more powerful tech can also lead to worker exploitation. But as the field evolves, new techniques are emerging that could reduce the need for human feedback. One such approach is the use of synthetic data. Rather than relying on armies of workers to assess training examples, AI models can learn from artificially generated data that mimics real-world patterns. For us down-stream consumers, as with many other globalised products, we shouldn’t forget what goes into the AI training supply-chain.
Takeaways: Tools like GPTZero aim to detect AI content by analysing things like perplexity and burstiness, but the technology is by no means reliable, and can put non-English speakers at a disadvantage by wrongly flagging their prose. In response, tools like StealthGPT are being developed to avoid such detection by better mimicking human writing patterns and flaws. Any business relying on AI for content generation will need to consider detection methods to avoid their material being increasingly flagged as inauthentic. Those wishing to validate the authenticity of information will need to understand and carefully employ equally cutting-edge detection.
Full transparency, this article was part generated by Claude 3 Opus. We tend to get help expanding an initial idea and provide Claude with lots of examples of our writing to ensure the style and perspectives are consistent. GPTZero suggested there was an 80% chance this was human written, which is likely down to the extensive edits we then make on the initially AI generated material.
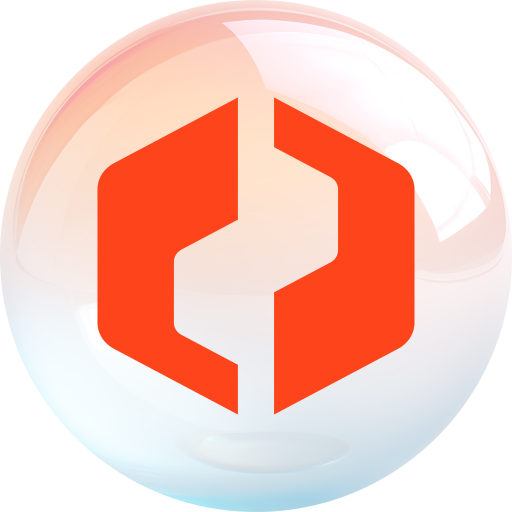
EXO
This week saw significant advancements in robotics, AI investment escalations, growing ethical and regulatory concerns about AI’s impact, and a focus on both hardware innovation and alternative architectures for accelerating AI progress.
AI business news
- Boston Dynamics’ new humanoid moves like no robot you’ve ever seen (This showcase of advanced robotics highlights the potential of AI to transform real-world tasks and automation.)
- Google will pump more than $100B into AI says DeepMind boss (This massive investment signifies the continued focus of major tech companies on AI development and competition.)
- LinkedIn testing Premium Company Page subscription with AI-assisted content creation (This feature demonstrates how AI tools are being integrated into professional platforms, streamlining content creation for businesses.)
- Adobe to integrate OpenAI’s Sora? (This integration demonstrates the accelerating adoption of AI in creative tools and hints at transformative potential in video editing.)
- Microsoft invests $1.5B in Abu Dhabi’s AI firm G42, gains board seat amid US-China tech tussle (This significant investment and partnership underscores the global competition for AI resources and talent.)
AI governance news
- Snap introduces watermarks for AI-generated images (This step aims to increase transparency about the use of AI in image generation, addressing the need for ethical AI practices.)
- UK government cracks down on ‘deepfakes’ creation (This crackdown reflects growing concern about the potential misuse of deepfakes and attempts to curb their harmful use.)
- GPT-4 can exploit real vulnerabilities by reading advisories (This finding further emphasizes the need for vigilance in AI safety and potential unintended consequences of advanced AI systems.)
- California’s Generative AI copyright disclosure act by Adam Schiff demands AI firms to disclose use of copyrighted material for transparency (This proposed legislation underscores the growing concern over intellectual property rights in the context of AI and the need for greater transparency.)
- Elections 2024: Tracking AI use in global elections – Rest of World (This site tracks the growing influence of AI in shaping political narratives and the challenges of combating AI-powered disinformation.)
AI research news
- ChatGPT can predict the future when it tells stories set in the future about the past (This study explores the unique capabilities of large language models and their potential for understanding temporal concepts.)
- VASA-1: Lifelike audio-driven talking faces generated in real rime (This research highlights advancements in AI-powered multimodal generation, potentially enabling more realistic virtual interactions.)
- Scaling instructable agents across many simulated worlds (This work explores techniques to enhance AI training in simulated environments, aiming to create more adaptable AI agents.)
- From words to numbers: Your large language model is secretly a capable regressor when given in-context examples (This research reveals hidden capabilities of large language models, expanding their potential applications.)
- A Survey on retrieval-augmented text generation for large language models (This paper provides an overview of research on enhancing language models with information retrieval, a topic we’ve discussed frequently.)
AI hardware news
- Another startup is taking on Nvidia using a clever trick – Celestial AI brings DDR5 and HBM together to slash power consumption by 90% (This innovation highlights competition in the AI hardware market and the pursuit of more efficient chip designs.)
- DoE receives Intel’s latest neuromorphic brain-in-a-box (This delivery signals investment in advanced hardware inspired by biological systems, which could lead to novel AI architectures.)
- TSMC expects Q2 sales to jump on ‘insatiable’ AI demand (This forecasted surge underscores the strong market demand for AI chips and the ongoing growth of the AI industry.)
- RISC-V AI chip startup Rivos nabs $250M in series A funding (This substantial investment demonstrates confidence in the potential of RISC-V as an alternative architecture for AI acceleration.)
- Intel to Launch HL-328, HL-388 AI chips in China with big performance drop (This launch, despite performance limitations, shows Intel’s efforts to navigate geopolitical tensions while participating in the growing AI market in China.)