Welcome to our weekly news post, a combination of thematic insights from the founders at ExoBrain, and a broader news roundup from our AI platform Exo…
Themes this week
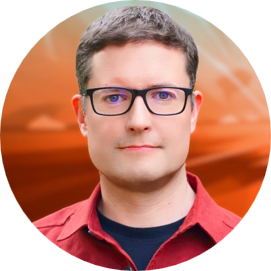
JOEL
This week we look at:
- How LLM’s maths capabilities are improving and what this means for the future, and for AGI.
- The political turmoil in the US, Trump’s VP pick and the implications for AI.
- New fast and cheap models, including GPT-4o Mini, that radically drive down the cost of intelligence.
Language models do the math
While LLMs are generally thought to struggle with mathematical tasks, news this week and in recent months suggest we’re witnessing some significant improvements in this area. Let’s face it, most humans are pretty bad at maths, but some show exceptional talent. It’s more or less the same with AI models, with both specialised and larger frontier models demonstrating improving capabilities. This evolution in mathematical reasoning isn’t just a technical milestone – it’s also potentially an accelerant on the path to artificial general intelligence (AGI).
Released this week, Mistral AI’s Mathstral, a 7B parameter model designed for STEM applications, has shown impressive results on the MATH benchmark (a dataset of 12,500 challenging competition mathematics problems) for a small open-weight model you can run on your laptop. Meanwhile, Harmonic’s Aristotle has been progressing on the MiniF2F, a benchmark for testing AI systems’ formal mathematical abilities. The developments aren’t limited to the smaller labs. Various rumours suggest that OpenAI have been internally demonstrating a model with powerful maths reasoning, while Google’s Gemini 1.5 Pro has been shown to achieve 91.1% on the MATH without tool-use, the most of any public model so far.
As we previously covered, Scale AI have been developing GSM1k, an entirely new set of problems mirroring the difficulty of the popular GSM8k benchmark, but where it’s not possible the models have been trained on these questions. An example of the kind of question posed is: “Gabriela has $65.00 and is shopping for groceries so that her grandmother can make her favourite kale soup. She needs heavy cream, kale, cauliflower, and meat (bacon and sausage). Gabriella spends 40% of her money on the meat. She spends $5.00 less than one-third of the remaining money on heavy cream. Cauliflower costs three-fourth of the price of the heavy cream and the kale costs $2.00 less than the cauliflower. As Gabriela leaves the store, she spends one-third of her remaining money on her grandmother’s favourite Girl Scout Cookies. How much money, in dollars, does Gabriela spend on Girl Scout cookies?” Today a frontier model like Claude 3 can answer 950 out of 1,000 of these questions that it hasn’t seen before, correctly.
Beyond intrinsic model capabilities, in the Artificial Intelligence Math Olympiad (AIMO) a team showcased a novel approach to enhancing LLMs’ ability on much harder problems, by combining structured thinking with code execution. The team from Numina and Hugging Face used high-quality instruction data for competition-level maths, then integrated this with code generation capabilities. This hybrid approach allowed their model to break down complex problems into steps, run Python code to reason about each stage, and ultimately achieve impressive performance gains. The technique not only improved accuracy but also reduced variance in solutions, demonstrating how creative combinations of existing methods can push boundaries.
But why does this matter beyond the world of AI researchers? Chinese lab DeepSeek’s Liang Wenfeng believes the path to AGI means betting on 3 areas: mathematics, multimodality, and language. “Mathematics and code are the natural testing grounds for AGI,” he notes, and a particularly important proving ground as maths is “a verifiable system that [can support] high intelligence through self-learning.”
Maths capability is also vital for machine-verifiable ‘proofs’. Harmonic’s Aristotle can take a natural language maths problem and translate it into a formal proof in ‘Lean 4’, a language for mathematical reasoning. This kind of process can help address a critical concern in AI adoption: trust. By producing formally verified proofs (autoformalization) models can show their workings out in a 100% verifiable way, crucial for deploying AI in critical applications like designing bridges or drugs, where we need to be 100% sure it’s not just guessing the answer. Proofs have many other uses, from cryptography, smart contracts, and hardware security to exploring new mathematical ideas.
Whilst maths is not a comfort zone for many, nor has it been for language-based AI, models are now rapidly becoming adept at tackling complex problems. This progress could democratise advanced mathematical reasoning, making it accessible to a broader range of industries and applications, and help strengthen AI’s value in many other areas.
Takeaways: For businesses and AI users, these developments should trigger the reassessment of AI’s potential in domains requiring complex reasoning. Mathematical capabilities can be highly effective for many business tasks. If a use-case hasn’t worked with a language-based approach, it may now be solvable with one that employs more logical reasoning. As AI maths evolves, staying informed and understanding how to exploit the power of mathematical proofs will be highly beneficial.
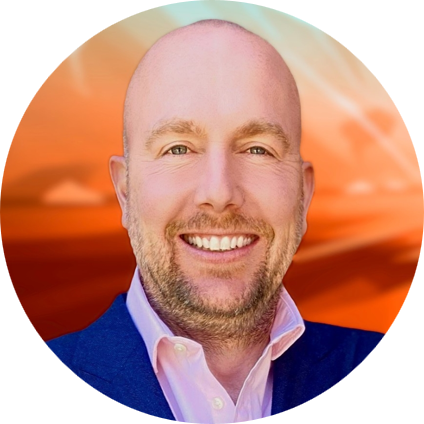
JOOST
Would Trump “Make America First in AI”?
The tumultuous 2024 US presidential race is setting the stage for a significant debate on AI policy and regulation. This week, conversations have been heating up in tech circles about how the contrasting approaches of leading candidates could reshape future trajectories.
Donald Trump’s selection of J.D. Vance as his running mate, dubbed a “tech bro ” on the ticket by some, hints at a potential alignment with Silicon Valley conservatives. This pairing could lead to policies favouring open-source advocates and possibly easing regulations. However, Trump’s vision may not be a purely unrestricted one. The Washington Post reports that a Trump aligned institute has drafted a “Make America First in AI” policy that would launch a series of “Manhattan projects” to increase the militarisation and protection of US AI capabilities. Moreover, Trump’s unpredictable approach to foreign policy could have significant implications for GPU supplies. His recent comments on Taiwan, urging the island to shoulder more of its defence costs, have already rattled financial markets and chipmaker stocks. And the polarising nature of Trump does not stop there, his personal relations could impact policy too. Trump’s long-standing feud with Meta’s Mark Zuckerberg adds a layer of complexity, with Zuckerberg’s firm an increasing AI powerhouse. The animosity could influence regulatory actions against specific social media platforms and impact their leverage in the race to more advanced systems.
In contrast, a Democratic victory would likely usher in a more regulated approach. Arati Prabhakar, the current Director of the Office of Science and Technology Policy, advocates for a balanced approach to tech regulation, focusing on both innovation and ethical standards. This could mean more comprehensive regulations aimed at ensuring technology serves the public good while mitigating risks. The implications for businesses and users of AI technology are significant. Companies may find themselves navigating a rapidly changing regulatory landscape, potentially affecting everything from product development to market strategies. Users could see changes in the speed of innovation and the level of protections against potential misuse or bias in AI systems.
The role of AI in the election itself is another critical factor. Both parties have expressed concerns about AI’s potential to create deepfakes and spread misinformation, highlighting the need for robust policies to protect election integrity. This presents both a challenge and an opportunity for AI companies to develop tools that can combat misinformation and enhance cybersecurity.
Takeaways: As the election approaches, businesses should prepare for potential regulatory shifts by developing flexible AI strategies. Users should stay informed about AI policies and their implications for privacy and rights. Policymakers and tech leaders must collaborate to strike a balance between innovation and regulation, ensuring AI serves the public good while maintaining national competitiveness in what is a global race. The outcome of this election could shape the trajectory of AI development and regulation for years to come, making it a crucial moment for the tech industry and society at large.
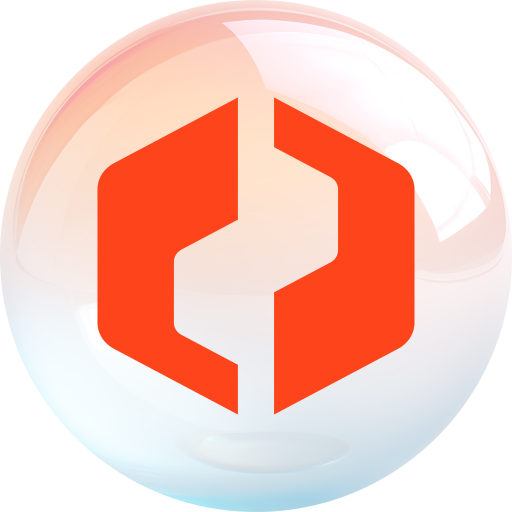
EXO
Intelligence too cheap to meter?
On Thursday OpenAI unveiled GPT-4o ‘Mini’, a scaled-down version of their most powerful model that’s 60% cheaper (around $0.24 per million blended tokens) than the old GPT-3.5. Once again, a lab is prioritising efficiency over raw intelligence and scale, to help maximise monetisation. It looks like the performance and cost will see it significantly undercut Google’s Gemini Flash and Anthropic’s Claude 3 Haiku, the previously leading low-cost options. Groq, the chip and inference provider also released new models including Llama-3-Groq-8B-Tool-Use, which will cost just $0.19 per million tokens, and can run at over 1,000 tokens per second. All in we’ve seen a staggering 100x reduction in cost for using AI models in only 2-years.
These developments highlight the ongoing race in the AI industry to balance performance and efficiency. As AI capabilities evolve, so does the computational power required to run these models, leading to significant environmental and economic concerns. The push for a more streamlined AI is driven by growing competition among providers and rising interest in smaller, specialised models that can perform specific tasks with high efficiency.
For ChatGPT this likely means the end of the road for GPT-3.5, the model that started it all when released back in 2022. For end-users, these advancements should translate into smarter and more responsive experiences across the board from chatbots to AI enabled apps. Longer term, how low can costs go? In a post on X promoting the launch, Sam Altman, CEO of OpenAI intimated that their goal was “intelligence too cheap to meter”?
Takeaways: As AI models become both more powerful and more efficient, businesses should constantly reassess their AI strategy. Consider the balance between specialised and general-purpose models in your stack and understand how new price points might unlock previously non-viable use-cases. You can test out GPT-4o Mini on ChatGPT Premium now.
Weekly news roundup
This week’s news highlights the growing impact of AI on various industries, increased focus on AI governance and security, advancements in AI research, and developments in AI hardware, particularly in chip manufacturing and data centre technologies.
AI business news
- AI could give the UK a productivity boost worth billions of pounds (This story underscores the potential economic impact of AI adoption, a recurring theme in our discussions about AI’s transformative power.)
- Samsung buys Oxford Semantic and its AI knowledge graph tech (This acquisition highlights the growing importance of knowledge graphs in AI, a topic we’ve explored in previous newsletters.)
- 5 ways Intel’s ‘AI everywhere’ is powering the 2024 Paris Olympics (This showcases the practical applications of AI in large-scale events, demonstrating how AI is becoming ubiquitous in various sectors.)
- Salesforce introduces autonomous AI customer service agent powered by Einstein (This development aligns with our previous discussions on AI’s role in enhancing customer experiences and automating business processes.)
- Former OpenAI and Tesla exec Andrej Karpathy dives into education with AI-native school (This initiative reflects the growing need for AI-focused education, a topic we’ve highlighted as crucial for future workforce development.)
AI governance news
- On AI, new UK gov’t to work on ‘appropriate’ rules for ‘most powerful’ models and beef up product safety powers (This development is significant for our readers interested in the evolving landscape of AI regulation and governance.)
- Protect AI warns of increasing security risks in open-source AI and ML tools (This warning highlights the ongoing challenges in balancing open-source innovation with security concerns in AI development.)
- Apple, Nvidia, Anthropic used thousands of swiped YouTube videos to train AI (This story raises important questions about data ethics and copyright in AI training, a recurring theme in our coverage of AI development practices.)
- Microsoft faces UK antitrust probe after hiring Inflection AI founders and employees (This probe reflects the growing scrutiny of big tech’s influence in the AI sector, a topic we’ve discussed in relation to market competition and innovation.)
- Meta won’t bring future multimodal AI models to EU (This decision highlights the impact of regulatory environments on AI deployment, a crucial consideration for our readers involved in global AI strategies.)
AI research news
- Microsoft’s new AI system ‘SpreadsheetLLM’ unlocks insights from spreadsheets, boosting enterprise productivity (This development showcases the practical applications of AI in everyday business tools, a trend we’ve been following closely.)
- AgentInstruct: toward generative teaching with agentic flows (This research aligns with our ongoing discussions about AI’s potential to revolutionise education and training methodologies.)
- SciCode: a research coding benchmark curated by scientists (This benchmark is significant for our readers interested in the intersection of AI and scientific research, a topic we’ve explored in previous issues.)
- Human-like episodic memory for infinite context LLMs (This research addresses the challenge of long-term memory in AI systems, a crucial aspect for developing more capable and human-like AI.)
- Mixture of a million experts (This paper presents a novel approach to scaling AI models, which could have significant implications for future AI architectures.)
AI hardware news
- Chip stocks drop on report US plans to tighten China curbs (This development highlights the geopolitical tensions affecting the AI hardware industry, a recurring theme in our coverage of the global AI landscape.)
- Your next datacenter could be in the middle of nowhere (This trend reflects the growing demand for AI computing power and its impact on data centre locations, a topic relevant to our discussions on AI infrastructure.)
- TSMC second-quarter profit beats expectations as AI chip boom continues (This news underscores the ongoing AI-driven demand in the semiconductor industry, a key factor in the development of AI technologies.)
- Google chief scientist Jeff Dean: AI needs ‘algorithmic breakthroughs,’ and AI not to blame for most of data center emissions increase (This perspective from a leading AI researcher provides insights into the future directions of AI development and its environmental impact.)
- The AMD Zen 5 microarchitecture: powering Ryzen AI 300 series for mobile and Ryzen 9000 for desktop (This announcement highlights the ongoing integration of AI capabilities into consumer hardware, a trend we’ve been tracking in our coverage of AI’s expanding reach.)