Welcome to our weekly news post, a combination of thematic insights from the founders at ExoBrain, and a broader news roundup from our AI platform Exo…
Themes this week
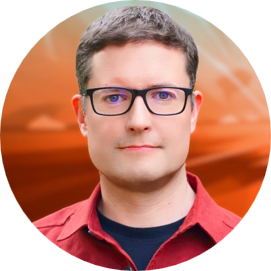
JOEL
Themes this week:
- UK Wayve’s $1 billion funding from Softbank and Nvidia drives the future of embodied AI in cars and robotics.
- Google DeepMind’s AlphaFold 3 borrows from image generation to unlock the secrets of proteins, DNA, and drug interactions.
- We explore how different industries are taking different paths to AI adoption.
Wayve takes a billion-dollar step towards embodying AI
Wayve, a UK-based autonomous vehicle (AV) startup, has just raised $1.05 billion in Series C funding, joining a select group of deep-tech unicorns that hail from these shores. This is significant news for the whole UK ecosystem, with PM Rishi Sunak expressing how “incredibly proud” he was of the investment. So, what makes Wayve special enough to secure one of the biggest funding injections of any AI firm outside of the likes of OpenAI and Anthropic?
Wayve are at the forefront of research on what’s called AV2.0 or the second generation of autonomous vehicle technologies. They use large neural nets, vision, and language models to help machines understand the world around them and take actions. Their latest on-board driving systems work in an ‘end-to-end’ way, directly mapping sensor inputs to driving outputs, via a large malleable AI model that runs all the capabilities once dealt with by many independent and inflexible subsystems. This end-to-end and trainable approach allows their system to adapt quickly to new environments and vehicles, making it efficient and scalable.
Wayve has also recently introduced something they call a ‘vision-language-action’ model that can understand sensor data and verbalise its driving and decision-making processes. They see this as a step towards building trust and transparency in autonomous vehicles, which will be crucial for full public acceptance. Wayve also use an advanced simulation environment called Ghost Gym to test and refine their systems. By generating synthetic data that covers a wide range of road use scenarios they can validate before testing on real vehicles. This is all being made possible by the convergence of ‘software-defined’ cars, advanced sensing, synthetic data, and of course- the power of (on-board) compute from none other than Nvidia.
Wayve reportedly garnered interest from automakers but opted for sector independence although they’re working with brands such as Jaguar and Ford. They believe the best AI will be built with data gathered from across the industry. CEO and co-founder of Wayve, Alex Kendall, explained that the funding will be used to curate and generate data and train ever more powerful models that deliver AI “we can trust to physically interact with us in the world”, but in the first instance to fully realise a product for the automotive market, although he is hesitant to give timelines.
Tesla, once the undisputed leader in electric and autonomous vehicles, is also exploring working with other automakers and licensing their Full Self-Driving (FSD). However, Tesla has had its share of challenges lately. FSD has had a chequered history, not least because it is not by any means a ‘full self-driving’ system. For many years it took an alternative route to Wayve and did not use deep learning beyond its image recognition functionality. But a switch to an end-to-end AI approach has seen it make significant strides of late.
Google’s Waymo spin-off continues to demonstrate impressive utility with its 250+ fleet of robo taxis operating in various US cities. At $200,000+ per vehicle, they are not yet commercially viable for mass adoption but have logged 7+ million miles in real-world conditions. They operate at level 4 autonomy, meaning they can handle most driving situations without human intervention, although they are still restricted to pre-defined geographical areas. For reference, level 1 is the kind of light assistance most of us have in our cars today and level 2 provides partial automation with the driver needing to remain engaged at all times (“FSD” is level 2 hence the controversy). Level 3 would see the driver ready to take control at any time, whereas level 5 is full autonomy in all conditions.
With Tesla planning to test FSD powered robotaxis in China and companies such as GM’s Cruise and Amazon’s Zoox developing their own services, many companies are betting on the rapid growth of shared autonomous mobility, where populations will increasingly rely on on-demand self-driving vehicles rather than owning personal cars.
While the potential benefits of this could be immense – improved safety, reduced emissions, optimized land use, and more – there are still challenges to overcome. Electricity and charging infrastructure remain a huge limiting factor. And public demand, trust, and acceptance are crucial, with the regulatory landscape playing a significant role. The UK seems to be positioning itself favourably in this regard, which could give Wayve an added edge. The Automated Vehicles Bill completed its journey through parliament this week, and is shaping the frameworks necessary for the marketing, insurance, safety, data sharing, and cyber security of self-driving systems.
Longer-term both Tesla and Wayve are working towards a vision of widespread ‘embodied AI’. They imagine a continuum from uses in AVs into other complex physical scenarios. The implications of embodied AI extend far beyond our transport systems. Tesla’s Optimus humanoid line was this week seen showing off its 22 degrees of freedom finger dexterity (5 short of a human’s 27), and Musk hopes it will be on sale by year end. Wayve’s platform for simulating, teaching, learning, explaining, and acting could be applied to many scenarios. From industrial robotics to healthcare assistants and space exploration, the full potential of embodied AI lies in its ability to enable machines to perceive, understand, and interact with the physical world in increasingly sophisticated ways ultimately reshaping our relationship with technology in every aspect of life.
Wayve’s $1 billion funding round is another indicator of the progress being made in AI applications beyond the chatbot. It’s also an indicator of commercial potential big tech firms see in taking a slice of a transportation pie that’s forecast to grow to $6 trillion by 2030. AVs was once (what venture capitalist Steve Vassallo) termed a “zero-billion-dollar” market, a market that didn’t exist, but had immense potential. Alongside AI agents, could embodied AI be the next zero-trillion-dollar market?
Takeaways: If you want to try a level 2 ‘hands-off eyes-on’ AV legally in the UK, the first car to be approved is the Ford Mustang Mach-E (2023 model) with BlueCruise. Ford state that 95% of the country’s motorways are designated hands-free Blue Zones, where it will manage steering, acceleration, and braking (for a £17.99/month subscription fee). This looks like the shape of things to come, with level 3 ‘eyes-off’ systems slated for legal approval in the UK from 2025/26. However, Waymo are not currently planning to deploy in the UK given the progress here is still somewhat behind the US and other countries. Where might you see the Wayve technology in action? Perhaps when the Asda or Ocado shopping arrives at your door, as both supermarkets are working towards trialling Wayve’s tech in their delivery fleets.
AlphaFold 3 further demonstrates AI’s transferability
London based Google DeepMind and Isomorphic Labs have announced Alpha Fold 3, the latest iteration of their groundbreaking AI system designed to predict the 3D structures of proteins — and now, much more.
Predicting protein structure from amino acid sequences has challenged scientists for decades, as function depends on shape. Solving this crucial problem accelerates everything from disease understanding to drug design. We wrote about the generation of genetic sequences back in week 9 with the Evo model, and now AlphaFold provides scientists with the ability to see how these fundamental structures of life actually interact, albeit only in static form at this stage.
AlphaFold 2 changed the game in 2020 by predicting protein structures with unprecedented accuracy. Thee system provided hundreds of thousands of protein structures for researchers worldwide, boosting studies in fields as diverse as genetics and biochemistry. This new version incorporates a diffusion model — a concept borrowed from AI systems designed to generate images like the pictures at the top of our news articles, and others created by the likes of Dall-E or Midjourney. These models work by gradually refining their guesses, starting from a randomly noisy image (or in this case, a molecular structure) to produce detailed and accurate pictures. In AlphaFold 3, this approach means the software can now model not just proteins but also DNA, RNA, antibodies, and even tiny molecules like metal ions. This ability to handle a broader range of biological molecules means AlphaFold 3 can predict how all these different entities interact. This is crucial for understanding complex biological processes and diseases at a molecular level.
AlphaFold 3’s enhanced capabilities allow researchers to see how a potential drug fits into a protein, how strong that interaction is, and what unintended targets the drug might also affect. This insight is invaluable for developing more effective and safer medications. The implications of these advancements are huge. For instance, by understanding how proteins interact with DNA, researchers can explore fundamental questions about cellular functions, such as how cells repair damaged DNA and how various diseases arise from these processes going awry.
Aside from the practical value, Google’s AI chief Demis Hassabis says could be worth north of $100 billion to the firm. While AlphaFold 3 is a significant step forward, it’s not without its open questions. DeepMind has previously been criticized for overhyping their scientific discoveries, as seen with their AI system called, Gnome (Graph Networks for Materials Exploration) which was purported to have discovered 380,000 new materials. This was subsequently challenged by experts for the lack of evidence provided on the utility and credibility of its newly predicted compounds. One other decision is that is likely to draw much criticism is that AlphaFold 3 will not be open-sourced. DeepMind has made it accessible for free via the cloud to researchers worldwide. This approach aims to balance the need for openness with the complexities of developing such advanced tools, and no doubt helps to manage the potential negative dual uses in creating dangerous pathogens or toxins. Anyone can try AlphaFold Server here.
Takeaways: The use of diffusion models in AlphaFold 3 illustrates the incredible versatility of modern AI. Diffusion can create photorealistic images from pixel noise, and it seems it can also figure out complex molecular structures from limited data. This echoes the re-usability of other key AI concepts such as vector embeddings, high dimensional numeric representations that allow systems to understand anything from words to sounds and pictures to robotic telemetry.
Dr Jim Fan, embodied AI lead at Nvidia posted on X: “We live on a timeline where learnings from Llama and Sora [OpenAI’s video model] can inform and accelerate life sciences. I find this level of generality absolutely mind-boggling. The same transformer+diffusion backbone that generates fancy pixels can also imagine proteins, as long as you convert the data to sequences of floats accordingly. We are not there yet at a single AGI [human-level] model, but we have successfully built a menu of general-purpose AI recipes that transfer training, data, and neural architectures across domains. This should not work, but thank god it does!”
This adaptability shows how AI can be a powerful tool across many domains, not just biology, by choosing the right approach for the right task, many things are possible.
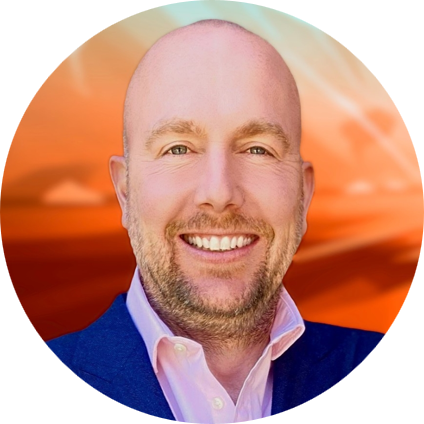
JOOST
Different paths to AI adoption for different industries
This week, several publications and articles looked at the impact of AI on various industries, revealing a growing recognition of its transformative potential, yet starting from different points. From asset management to journalism and creative industries, AI is reshaping the way businesses operate and deliver value to their customers, yet all at different speeds.
A BCG study found that 72% of surveyed asset managers believe AI will have a significant or transformative impact on their organization within the next three to five years, with 66% making AI a strategic priority. On the outset, these numbers feel low for an industry that faces an ever-increasing fee-pressure coupled with an increasing cost-base. AI is already being used to improve Asset Liability Management (ALM) and Strategic Asset Allocation (SAA) processes, leading to more efficient risk-adjusted returns and cost reductions of 5% to 15%. However, most asset managers are still in the early stages of developing their AI strategies, and investments in people, technology infrastructure, and risk management are lagging behind.
The asset management remains largely undisrupted and heavily regulated, so is understandably slow to get off the mark.
A different perspective comes from an industry that had its business model already fundamentally disrupted in the past. The FT expresses a more fearful outlook on the impact of AI on its business, journalism, drawing parallels to the (traumatising) dot.com boom. They argue that the widespread adoption of AI could significantly disrupt traditional news business models and revenue streams. If readers increasingly turn to AI-powered tools for news summaries, analysis, and Q&A, it may reduce direct traffic to news websites and apps. This in turn would impact subscription growth and ad revenue.
News organizations are forced to rethink their value proposition, again – focusing more on original reporting, deep subject matter expertise, and building direct audience relationships that AI aggregators can’t easily replicate. They may also need to develop their own AI offerings and tools to enhance the user experience and maintain engagement.
Other industries see and feel different urgencies to embrace AI.
TV production companies like RTL Group and Banijay are exploring AI-assisted content (and even format!) creation. While human creativity remains paramount, these companies recognize the potential of AI to contribute to genre defining entertainment. Eline van Der Velden, founder and CEO of Particle 6, believes that AI can help producers speed up processes and enabling them to focus on the creative aspects of their work. However, some industry experts, like Dan Whitehead from K7 Media, caution against relying too heavily on AI, arguing that it cannot replicate the human spark that often leads to hit shows.
We’ve seen significant human resistance in this industry (Hollywood writers’ strike), yet time will tell if AI-drive production companies and studios will see dramatic productivity increases at a much reduces cost-base.
Takeaways: The path to AI adoption will be different for every organisation. Each approach is driven by past experiences, risk appetite, willingness to change, limitations (regulatory), market drivers and many, many more factors. Although some organisations still doubt the disruptive ability of AI, there is one thing every organisation can and should do: Start small. Start now. Experiment.
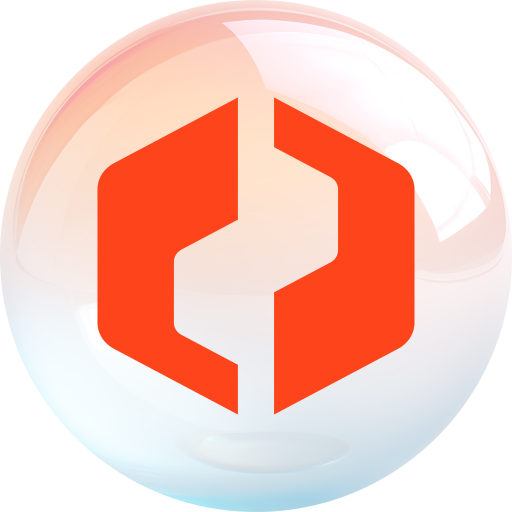
EXO
AI business news
- Stack Overflow signs deal with OpenAI to strengthen models on its data (Demonstrates how major tech platforms are partnering with AI companies to enhance their offerings and leverage AI capabilities.)
- iPad Pro’s new M4 chip boasts ’10x’ performance boost, faster AI (Highlights the growing integration of dedicated AI hardware in consumer devices, a trend we’ve discussed before.)
- OpenAI is entering the search game (Signals OpenAI’s ambitions to compete with major tech companies in the lucrative search market, leveraging its AI capabilities; look out for a big announcement on Monday…)
- ‘Use of generative AI has nearly doubled in the last six months, with 75% of global knowledge workers using it’ – Microsoft/LinkedIn survey (Underscores the rapid adoption of AI tools in the workplace, a theme we’ve highlighted previously.)
- Microsoft is ‘turning everyone into a prompt engineer’ with new Copilot AI features (Demonstrates how AI is becoming more accessible and integrated into everyday software tools, empowering users to leverage its capabilities.)
AI governance news
- OpenAI posts Model Spec revealing how it wants AI to behave (Sheds light on OpenAI’s efforts to ensure responsible AI development and align their models with desired behaviours, a topic we’ve discussed frequently.)
- OpenAI to launch tool to detect images created by DALL-E 3 (Addresses the growing concern around deepfakes and the need for tools to distinguish AI-generated content, a theme we’ve explored before.)
- Met Gala AI photos of Katy Perry, Rihanna and Dua Lipa trick the internet (Highlights the potential for AI-generated content to spread misinformation, reinforcing the need for responsible AI governance.)
- ‘A chilling prospect’: should we be scared of AI contestants on reality shows? (Raises ethical questions about the use of AI in entertainment and the potential implications for societal norms and human agency.)
- Warren Buffet voices AI fears, likens tech to the atom bomb (Echoes the growing concerns from influential figures about the potential risks and unintended consequences of advanced AI systems.)
AI research news
- Prometheus 2: An Open Source Language Model Specialized in Evaluating Other Language Models (Highlights ongoing research efforts to develop AI systems capable of evaluating and analysing other AI models, a crucial component for responsible AI development.)
- SWE-AGENT: Agent computer interfaces enabling automated software engineering (Demonstrates the potential of AI to revolutionise software development, an area we’ve discussed as a promising application of AI technology.)
- AlphaMath Almost Zero Enhances LLMs with Monte Carlo Tree Search (MCTS) to improve mathematical reasoning capabilities (Showcases advancements in enhancing the reasoning and problem-solving abilities of language models, a key area of AI research.)
- Chain of thought empowers transformers to solve inherently serial problems (Highlights research into improving language models’ ability to tackle complex, multi-step problems, a critical capability for more advanced AI systems.)
- Inventor of LSTM unveils new architecture for LLMs to replace transformers: xLSTM (Signals potential breakthroughs in AI model architectures, which could lead to more powerful and efficient language models.)
AI hardware news
- Apple developing AI data centre chips to rival NVIDIA, will also use M2 (Underscores the growing competition in the AI chip market as major tech companies invest in custom hardware to support their AI ambitions.)
- AI in hardware market to expand at a robust 26.96% CAGR through 2031 (Highlights the projected growth and increasing importance of specialized AI hardware, a trend we’ve discussed previously.)
- US chip manufacturing capacity projected to triple by 2032, fuelled by CHIPS Act (Emphasizes the US government’s efforts to boost domestic chip production, essential for supporting the growing AI industry and addressing geopolitical tensions.)
- SoftBank in advanced talks to buy UK AI chip designer Graphcore (Signals continued investment and acquisition activity in the AI hardware space, as companies compete to develop cutting-edge solutions.)
- US revokes licences for sales of some chips to China’s Huawei (Highlights the ongoing geopolitical tensions surrounding AI hardware and the impact of trade restrictions on the global AI landscape.)