Welcome to our weekly news post, a combination of thematic insights from the founders at ExoBrain, and a broader news roundup from our AI platform Exo…
Themes this week
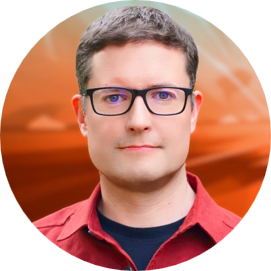
JOEL
This week we cover music’s turn in the AI spotlight, the challenges of AI adoption, and a deluge of new and updated models:
- Udio v Suno (v Spotify) fight! But what does this mean for the creative industries?
- AI has an adoption problem.
- Harder, better, faster, stronger… the fruits of massive investment in foundation models continue to deliver.
Udio sets a new benchmark in music generation
This week the launch of the new music generation service Udio put AI’s impact on music and the creative world front and centre. Services such as Udio (from will.i.am and some former Google DeepMind researchers), Suno or Stability AI’s recently launched Audio are giving people with the tools to dynamically create high quality music in any genre, both with generated or provided lyrics or in instrumental form. Meanwhile Spotify, now somewhat the old guard, adopt AI through their prompt based playlist generation tool. Udio has impressed with its notably higher levels of audio fidelity and fluency, although there is still some way to go for these services to replicate the clarity and impact of a professionally prepared track. Its also interesting to note that humans seem much more sensitive to audio anomalies than those found in other forms of generation. But much like with text, image and video the progress is rapid, we are probably no more than a year away from creative works that can be generated in any medium that are indistinguishable from professional output.
Before we get onto the wider reflection, a quick word on the tech. Suno, and likely Udio, are re-using language (LLM) architecture, but they’re feeding in fragments (or tokens) of audio not text. These models are trained on lots of ‘tokenised’ musical sequences (no doubt meaning more battles over copyrighted materials) and they teach themselves to predict tokens that could come next. This approach seems to be working for everything… From video, to music, to DNA, and even sensor data and robotic movements (see our research news)… chop up a pattern, feed it into a giant neural network and hey presto. And the bigger the neural network, the more compute, the better the output.
The economic and scientific impacts of this tech are driving a productivity revolution. But creativity and productivity are not the same. And our evaluation and consumption of creative outputs are fundamentally different from the way we treat the fruits of industrial transformation. Music in particular has unique social value; it can induce powerful emotional responses, physically synchronises the brain activity of live audiences, helps us form life-long identities, is bound up in our memories, and plays central roles in our rituals and community. It has a precious and finite value. And we humans are absolutely hard wired to appreciate its scarcity. We signal our status by demonstrating our exclusive access, and we manufacture scarcity by assigning attributes that create rarity or personal significance. Plus young and old we can’t seem to get enough of the excitement generated a concentrated group of cultural icons. The recording and streaming tech revolutions of the 20th and 21st centuries already changed music’s unit economics but these human and social fundamentals did not and will not change. We may come to deify AI artists… Claude has a controversial side that is already building a devout following in the world of text performance (more on that next week)… but whether AI, human or human augmented music superstars, we’ll tune out the noise to maintain the scarcity.
The music industry as we know it is likely to continue to grow, for now. But the music industry is a ~10th the size of the gaming industry. Our linear, time bound music consumption limits the amount of music that we consume. Perhaps we can look to younger generations, who are increasingly combining their consumption of music, video and gaming experiences in fragmented non-linear ways. Multi-modal AI generation could inspire decentralised, globally networked but hyper-personal open worlds. Curation, scarcity, rarity, connection, and meaning may be infinitely shaped and transmitted in new forms of interactive play. As with language models, music generation models will get open-sourced and become easy to run on your own machine, to train on your own musical tastes. The creation and sharing of music in this form will be less a centralised industry and more a new interactive entrainment medium, and that is likely the only path to a workable business model for these new services.
As Sam Altman teased this week (from his self-appointed position on the other side of the looking glass); “movies are going to become video games and video games are going to become something unimaginably better”. Perhaps music will also evolve into new forms, we’ll see an expansion of what is possible, rather than a replacement for the current music industry. Suno and Udio are part revolutionary, part conventional first experiments on the journey to an ever more decentralised and fluid creative landscape.
Takeaways: Here’s an uplifting ExoBrain rap… You can draw your own conclusions on whether the world needs more or less AI innovation hip hop, but Udio’s ability to generate believable music is undoubtedly impressive.
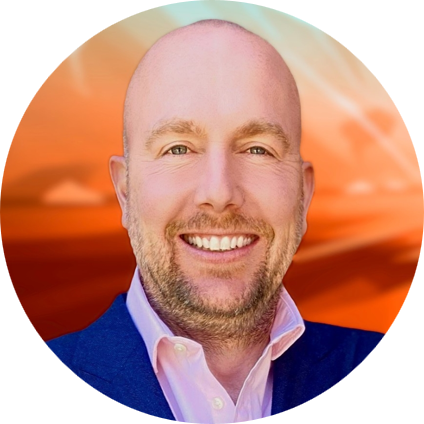
JOOST
AI has an adoption a problem
In the early 1900s, Mary Anderson, a serial inventor from Alabama, was traveling on a streetcar in New York City when she noticed a problem. The driver repeatedly stopped to clear snow and sleet from the windscreen, making the journey slow and dangerous. Inspired, Mary designed the first windscreen wiper in her small workshop.
With cars not yet widely adopted, Mary pitched her invention to local public transportation operators in snowy cities. However, they swiftly dismissed her idea as unnecessary and impractical, believing that drivers could continue clearing windscreens manually, as they had always done.
It wasn’t until years later, when the car industry began to boom, that wipers became a standard feature on cars. By this time, Anderson’s patent on her invention had expired, and she failed to capitalise on her new technology. Anderson faded into obscurity, and her role in the history of the automobile was largely forgotten.
How different Mary’s opportunity would have been if cars had been mass-produced already, if the local operators had been able to think outside the existing paradigm, or if her clients had been able to implement the new technology to improve the efficiency and effectiveness of their products.
JPMorgan’s Jamie Dimon highlighted in his annual letter to shareholders this week, that AI could be as transformational as some of the major technological inventions of the past several hundred years, likening it to the printing press, the steam engine, electricity, computing, and the Internet etc.
A survey this week by Adecco and Oxford Economics reveals that while 61% of executives believe AI is a game changer for their industry – with even higher percentages in the tech and automotive sectors – 57% lack confidence in their leadership team’s AI skills and knowledge.
Just as Mary Anderson’s clients failed to recognize the potential of her windscreen wiper invention, many businesses today risk missing out on the transformative power of AI as they struggle to understand its potential, where it will integrate into their organisations and what skills and knowledge are required to do so. We are on the boundary of an amazing opportunity, yet very few seem to know where to stick the windscreen wiper.
As Professor Ethan Mollick (author of Co-Intelligence: Living and Working with AI) tweeted this week: “A thing that I keep seeing in organizations I talk to: a few senior people are experimenting with AI in their work and realizing that this is going to be a huge deal in their industry…. yet they have huge trouble getting their colleagues to even try the systems out seriously.”
AI is serving up potential competitive advantage for companies; every week we cover real-world examples of how AI is surpassing human capabilities and disrupting industries; it’s music this week, it was software development with “Devin” a few weeks ago. So, with this in mind, how do the vast number of business respond to this transformative tech?
Current they don’t. AI has an adoption problem. A big mismatch between opportunity and implementation.
Yes, many companies have implemented low-level chatbot or meeting note automation tool. The real value of AI to gain competitive advantage does not lie in the periphery of personal efficiency enhancements. It will lie in incorporating additional, superior intelligence into the core value streams, your combined set of activities that add value to your customers.
Takeaways: AI has an adoption problem, but unlike the past, we don’t have the luxury to watch from the sidelines and wait for others to solve it. AI is here. Go use it. Now.
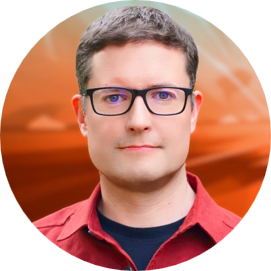
JOEL
A wave of new model announcements
It was a super busy week in AI models; this is likely to be a long-running theme as big tech dollars continue to pour into foundational development.
As a reminder where the name includes “7b” or “32b” etc, this is the number of parameters or virtual ‘neurones’ in the model’s brain in billions. For reference GPT-4 is rumoured to be 16x111b which means its got a total of 1.77 trillion parameters. As we’ll find out this week many of the latest models achieve near it’s levels of capability with a lot less:
- Canadian outfit Coherer’s Command R+ 104b model jumped up the leader boards this week. Fast, capable and flexible, Cohere have a winner on their hands, especially for the enterprise market. (At least for this week.) It’s also possible to run this on a powerful Mac or PC with 64GB+ of RAM. Cohere have a brilliant AI toolkit you can try out here.
- Google’s Gemini Pro 1.5 with 1 million token input window became generally available in API form to start powering new products. It’s audio ingestion is particularly impressive, but the big 1 million inputs will not be cheap.
- OpenAI made sure to steal some thunder from Google by releasing their news just hours later. They finally shipped something of real note, although their release management was somewhat chaotic (there isn’t even a blog post to link to, no release notes, and nothing from Sam Altman on what is seemingly a key launch for the under-pressure lab). GPT-4 Turbo has been enhanced and the rumour mill theorised it has had the benefit of being fine-tuned on the output of an unreleased and more powerful sibling eg GPT-5? Initial evidence suggests it’s better at coding and maths. The new version is now available in ChatGPT.
- Almost simultaneously Mistral uploaded a giant new open open-weight model to the Internet, making a torrent available for anyone to grab. It’s a ‘Mixtral’ 8x22b, a super-efficient mixture of experts (an intelligent bundle of smaller brains that work together, 8x indicating the number of combined experts being 8) that will give many bigger models a run for their money but will be highly cost effective.
- JetMoE 8b is another open mixture of experts model that caught the headlines this week due to details revealed about its training budget. It reportedly burnt up a mere $100k, that’s significant because it’s a Llama 2 7b class model, which a year ago cost Meta multiple millions to train.
- Meanwhile we saw Alibaba release Qwen 1.5 32b, to fill the gap between small and larger open models. Google added further diversity to the open weight arena by releasing some Gemma variants, and embattled Stability AI launched a bigger LM2 language model. Finally Universal-1 is a new best in class speech-to-text model from Assembly AI.
- Next week won’t be any quieter with the much anticipated arrival of some small Meta Llama 3 models that will provide us with the first indications of the next wave of capability.
Takeaways: There are 2 notable signals in this barrage of release noise. The ability to run a high-end model on a laptop with Command-R+, and the JetMoE cost of just $100k for a powerful AI training run. All the way back in 2023 you needed tens of millions and a vast data centre to create any kind of serious AI. Just 1-year on some MacBook Pros and a departmental budget will be more than enough.
Check out our research section below for some of the ideas that will be powering the next wave of models, with infinite inputs, new modes of learning, and architectures.
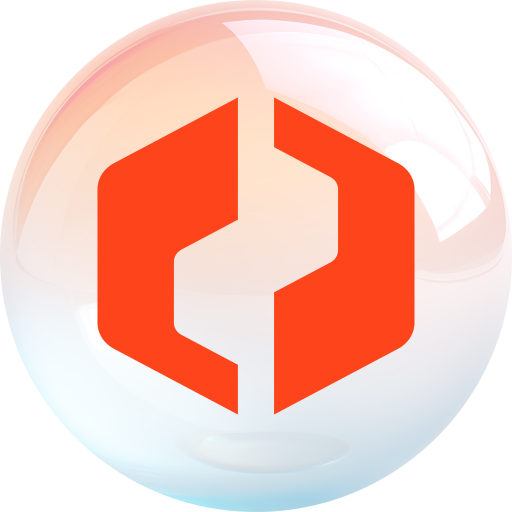
EXO
This week highlights intense competition for AI dominance, with massive investments in talent, data, hardware, and regulation, all pointing towards a future where AI will become even more integral to the global economy.
AI business news
- Microsoft to launch major AI hub in London (This expansion signals growing investment in AI on a global scale and emphasizes the focus on AI talent centres.)
- AI is helping us work up to 41% more effectively, according to recent Bain research. FlexOS reviews the platforms to consider (This research highlights the real-world impact AI is having on productivity and showcases tools businesses can use to leverage AI.)
- Intel CEO predicts AI will deliver a one-person Unicorn (This prediction emphasizes the potential of AI to empower individuals and disrupt traditional business models.)
- AI hardware company from Jony Ive, Sam Altman seeks $1 billion in funding (This funding round draws attention to the intersection of high-profile figures and cutting-edge AI hardware development.)
TikTok is testing an AI feature that generates a virtual influencer and a script for a video ad based on prompts submitted by advertisers (This highlights the growing use of AI in advertising and the potential for AI-generated content to compete with human creators.)
AI governance news
- OpenAI transcribed over a million hours of YouTube videos to train GPT-4 (This underscores the scale of data used to train powerful AI models and raises potential copyright concerns, a topic we’ve discussed before.)
- Bill would require AIs to reveal copyrighted training data (This proposed legislation addresses the issue of transparency in AI training and aims to protect intellectual property.)
- UK competition watchdog has ‘real concerns’ over big tech AI dominance (This highlights the growing scrutiny faced by major tech companies regarding their dominance in the AI space and potential anti-competitive practices.)
- Centre for AI Policy proposes the Responsible Advanced AI Act which would require US firms to obtain ‘frontier AI permits’ (This proposal demonstrates efforts to establish regulatory frameworks for advanced AI systems, ensuring their safe and responsible development.)
- Anthropic says its Claude model can change people’s minds (This claim highlights the potential of AI in persuasion and communication, prompting ethical considerations.)
AI research news
- Leave No Context Behind: Efficient Infinite Context Transformers with Infini-attention (This research expands the capabilities of AI models to handle vast amounts of information, potentially enhancing their performance.)
- Unsegment Anything by Simulating Deformation (This novel approach to image segmentation could lead to breakthroughs in AI-powered image and video analysis.)
- A neural speech decoding framework leveraging deep learning and speech synthesis (This framework suggests advancements in AI’s ability to understand human speech via the brain.)
- THOUGHTSCULPT: Reasoning with Intermediate Revision and Search (This research explores ways to improve AI’s reasoning and problem-solving abilities.)
- Keypoint Action Tokens Enable In-Context Imitation Learning in Robotic movement (This technique could accelerate the development of robots that can learn from human demonstrations.)
AI hardware news
- Statement from President Joe Biden on CHIPS and Science Act Preliminary Agreement with TSMC (This agreement highlights government support for domestic chip manufacturing and its importance in fuelling the AI industry.)
- Intel announces a chip that beats Nvidia H100 (This development signals intensified competition in the AI chip market, potentially leading to more powerful and accessible hardware.)
- Meta unveils its newest custom AI chip as it races to catch up (This shows Meta’s commitment to developing specialized AI hardware to support its own AI initiatives.)
- Two ex-Googlers launch AI chip startup MatX (ex-Googlers launch AI chip, betting on the demand for AI-optimized hardware to challenge industry giants like Nvidia.)
- Google Cloud’s AI Hypercomputer cloud infrastructure gets new GPUs, TPUs, optimized storage and more (This expansion underscores the ongoing investment in AI-focused cloud infrastructure.)